New machine learning model improves prediction of prostate cancer recurrence
Philadelphia | February 14, 2023
Machine learning model combining the expression of fusion genes, serum PSA level, and Gleason score consistently predicts the recurrence of prostate cancer more accurately than clinical data alone, improving patient care, investigators report in The American Journal of Pathology
Predicting the course of prostate cancer is challenging because only a fraction of prostate cancer patients experience recurrence after radical prostatectomy or radiation therapy. Yet, prostate cancer remains one of the most fatal malignancies in men in the United States. Now, investigators have developed a machine learning model that combines profiles of fusion genes known to be widespread in prostate cancer with the commonly used Gleason score and prostate-specific antigen (PSA) level. The machine learning model consistently improved the prediction of prostate cancer recurrence by the clinical tests alone or in combination. The results opens in new tab/window are reported in The American Journal of Pathology opens in new tab/window, published by Elsevier.
“Gleason score and PSA level have been used with varying success in predicting clinical outcomes in patients with prostate cancer,” explained lead investigator Jian-Hua Luo, MD, PhD, Department of Pathology, University of Pittsburgh School of Medicine, Pittsburgh, PA, USA. “However, they provide limited insight into the mechanism of the disease. Gene fusion events are known to be widespread in prostate cancer, but their potential in predicting the course of the disease was unknown.”
Data from a multi-institutional cohort that included 271 samples of radical prostatectomy from the University of Pennsylvania Medical Center (UPMC), 191 from University of Wisconsin–Madison, and 112 from Stanford Medical Center were analyzed. All 14 of the fusion genes known to be present in prostate cancer were detected in the samples from the combined cohort. Gleason and serum PSA scores were also available.
The investigators first developed a training model using the UPMC data. Several machine learning algorithms were applied to the fusion gene profiling data to determine the best parameters of 14 fusion gene combinations for predicting prostate cancer recurrence. The best algorithms were then applied to the whole training cohort to build a model.
Prediction of cancer recurrence based on Gleason score alone had 77.9% accuracy, and PSA alone correctly predicted 73.5% of prostate cancer recurrence. When the Gleason score data were incorporated in the machine learning analysis with the fusion data, a total of 442 models of different combinations showed an accuracy above 80% for the combined models. When PSA alone was combined with fusion data, 265 models of different combinations showed prediction rates above 75%. The combination of fusion data, Gleason score, and PSA improved the prediction of prostate cancer; 317 models yielded prediction rates of 80% or better.
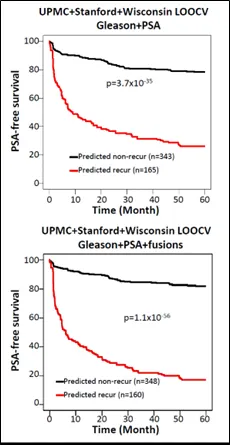
Caption: Fusion gene-containing algorithms enhance PSA-free survival prediction by Gleason score, serum PSA level, or the combination of both in the combined cohorts of UPMC, Stanford, and Wisconsin (Credit: The American Journal of Pathology).
Next, 764 machine learning models trained using data from the UPMC cohort were applied to the Stanford/Wisconsin cohort, and then to the UPMC/Stanford/Wisconsin cohort. Again, the combination of fusion data, Gleason score, and PSA outperformed the prediction of cancer recurrence by PSA or Gleason score alone or combined. Cancer did not recur for five years after surgery in 81.9% of patients if the cancer was predicted as nonrecurrent, while only 17.2% of patients were recurrence free if their cancer was predicted as recurrent by the same model. With the Gleason plus PSA model, 78.3% of patients had no cancer recurrence if the cancer was predicted as nonrecurrent by the model, and 26.2% of patients had no cancer recurrence for five years if the cancer was predicted as recurrent.
Dr. Luo notes that profiles of fusion gene have added value for clinical patient management because some gene fusions are important molecular processes in generating prostate cancer, whereas others are known to make cancer susceptible to certain drugs. “One of the big surprises in the analysis is that the fusion genes called CCNH-C5orf30 turned out to be an indicator of favorable clinical outcomes. It is unusual for a genomic abnormality created by cancer cells to restrain cancer’s aggressiveness,” he said.
“The detection of fusion genes provides new mechanistic insight into prostate cancer progression enabling proactive measures to be taken,” Dr. Luo observed. “The incorporation of fusion gene detection into the prostate cancer diagnostic scheme benefits patients with regard to diagnosis, prognosis, cancer progression surveillance, and treatment. Further, if these machine learning models are applied to clinical practice in the future, more lives may be saved.”
---
Notes for editors
The article is “Fusion Gene Detection in Prostate Cancer Samples Enhances the Prediction of Prostate Cancer Clinical Outcomes from Radical Prostatectomy through Machine Learning in a Multi- institutional Analysis,” by Yan-Ping Yu, Silvia Liu, Bao-Guo Ren, Joel Nelson, David Jarrard, James D. Brooks, George Michalopoulos, George Tseng, and Jian-Hua Luo (https://doi.org/10.1016/j.ajpath.2022.12.013 opens in new tab/window). It appears online in advance of The American Journal of Pathology, volume 193, issue 4 (April 2023), published by Elsevier.
The study was funded partly by NIH National Cancer Institute grant 1R56CA229262-01, US Department of Defense grant W81XWH-16-1-0541, and NIH National Institute of Diabetes and Digestive and Kidney Diseases grant P30-DK120531-01.
Full text of the article is available to credentialed journalists upon request. Contact Eileen Leahy at +1 732 238 3628 or [email protected] opens in new tab/window to request a PDF of the article. To request an interview with the authors please contact Jian-Hua Luo, MD, PhD, at +1 412 648 8791 or [email protected] opens in new tab/window.
About The American Journal of Pathology
The American Journal of Pathology opens in new tab/window, official journal of the American Society for Investigative Pathology opens in new tab/window, published by Elsevier, seeks high-quality original research reports, reviews, and commentaries related to the molecular and cellular basis of disease. The editors will consider basic, translational, and clinical investigations that directly address mechanisms of pathogenesis or provide a foundation for future mechanistic inquiries. Examples of such foundational investigations include data mining, identification of biomarkers, molecular pathology, and discovery research. High priority is given to studies of human disease and relevant experimental models using molecular, cellular, and organismal approaches. https://ajp.amjpathol.org opens in new tab/window
About Elsevier
As a global leader in scientific information and analytics, Elsevier helps researchers and healthcare professionals advance science and improve health outcomes for the benefit of society. We do this by facilitating insights and critical decision-making with innovative solutions based on trusted, evidence-based content and advanced AI-enabled digital technologies.
We have supported the work of our research and healthcare communities for more than 140 years. Our 9,500 employees around the world, including 2,500 technologists, are dedicated to supporting researchers, librarians, academic leaders, funders, governments, R&D-intensive companies, doctors, nurses, future healthcare professionals and educators in their critical work. Our 2,900 scientific journals and iconic reference books include the foremost titles in their fields, including Cell Press, The Lancet and Gray’s Anatomy.
Together with the Elsevier Foundation opens in new tab/window, we work in partnership with the communities we serve to advance inclusion and diversity in science, research and healthcare in developing countries and around the world.
Elsevier is part of RELX opens in new tab/window, a global provider of information-based analytics and decision tools for professional and business customers. For more information on our work, digital solutions and content, visit www.elsevier.com.
Contact
EL
CCP
Chhavi Chauhan, PhD
Director of Scientific Outreach
The American Journal of Pathology
+1 240 283 9724
E-mail Chhavi Chauhan, PhD