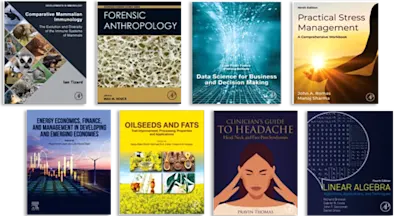
Algorithmic Trading Methods
Applications Using Advanced Statistics, Optimization, and Machine Learning Techniques
- 2nd Edition - September 4, 2020
- Imprint: Academic Press
- Author: Robert Kissell
- Language: English
- Paperback ISBN:9 7 8 - 0 - 1 2 - 8 1 5 6 3 0 - 8
- eBook ISBN:9 7 8 - 0 - 1 2 - 8 1 5 6 3 1 - 5
Algorithmic Trading Methods: Applications using Advanced Statistics, Optimization, and Machine Learning Techniques, Second Edition, is a sequel to The Science of Algorithm… Read more
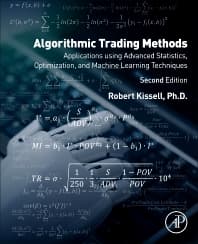
Purchase options
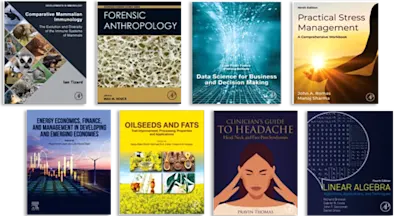
Institutional subscription on ScienceDirect
Request a sales quoteAlgorithmic Trading Methods: Applications using Advanced Statistics, Optimization, and Machine Learning Techniques, Second Edition, is a sequel to The Science of Algorithmic Trading and Portfolio Management. This edition includes new chapters on algorithmic trading, advanced trading analytics, regression analysis, optimization, and advanced statistical methods. Increasing its focus on trading strategies and models, this edition includes new insights into the ever-changing financial environment, pre-trade and post-trade analysis, liquidation cost & risk analysis, and compliance and regulatory reporting requirements. Highlighting new investment techniques, this book includes material to assist in the best execution process, model validation, quality and assurance testing, limit order modeling, and smart order routing analysis. Includes advanced modeling techniques using machine learning, predictive analytics, and neural networks. The text provides readers with a suite of transaction cost analysis functions packaged as a TCA library. These programming tools are accessible via numerous software applications and programming languages.
- Provides insight into all necessary components of algorithmic trading including: transaction cost analysis, market impact estimation, risk modeling and optimization, and advanced examination of trading algorithms and corresponding data requirements
- Increased coverage of essential mathematics, probability and statistics, machine learning, predictive analytics, and neural networks, and applications to trading and finance
- Advanced multiperiod trade schedule optimization and portfolio construction techniques
- Techniques to decode broker-dealer and third-party vendor models
- Methods to incorporate TCA into proprietary alpha models and portfolio optimizers
- TCA library for numerous software applications and programming languages including: MATLAB, Excel Add-In, Python, Java, C/C++, .Net, Hadoop, and as standalone .EXE and .COM applications
Upper-division undergraduates, graduate students, researchers, and professionals working in financial economics, especially trading
- Cover image
- Title page
- Table of Contents
- Copyright
- Preface
- Acknowledgments
- Chapter 1. Introduction
- What is Electronic Trading?
- What is Algorithmic Trading?
- Trading Algorithm Classifications
- Trading Algorithm Styles
- Investment Cycle
- Investment Objective
- Information Content
- Investment Styles
- Investment Strategies
- Research Data
- Broker Trading Desks
- Research Function
- Sales Function
- Implementation Types
- Algorithmic Decision-Making Process
- Chapter 2. Algorithmic Trading
- Advantages
- Disadvantages
- Growth in Algorithmic Trading
- Market Participants
- Classifications of Algorithms
- Types of Algorithms
- Algorithmic Trading Trends
- Day of Week Effect
- Intraday Trading Profiles
- Trading Venue Classification
- Types of Orders
- Revenue Pricing Models
- Execution Options
- Algorithmic Trading Decisions
- Algorithmic Analysis Tools
- High Frequency Trading
- Direct Market Access
- Chapter 3. Transaction Costs
- What are transaction costs?
- What is best execution?
- What is the goal of implementation?
- Unbundled Transaction Cost Components
- Transaction Cost Classification
- Transaction Cost Categorization
- Transaction Cost Analysis
- Implementation Shortfall
- Implementation Shortfall Formulation
- Evaluating Performance
- Comparing Algorithms
- Independent Samples
- Median Test
- Distribution Analysis
- Chi-Square Goodness of Fit
- Kolmogorov–Smirnov Goodness of Fit
- Experimental Design
- Final Note on Posttrade Analysis
- Chapter 4. Market Impact Models
- Introduction
- Definition
- Derivation of Models
- I-Star Market Impact Model
- Model Formulation
- Chapter 5. Probability and Statistics
- Introduction
- Random Variables
- Probability Distributions
- Probability Distribution Functions
- Continuous Distribution Functions
- Discrete Distributions
- Chapter 6. Linear Regression Models
- Introduction
- Linear Regression
- Matrix Techniques
- Log Regression Model
- Polynomial Regression Model
- Fractional Regression Model
- Chapter 7. Probability Models
- Introduction
- Developing a Probability Model
- Solving Probability Output Models
- Examples
- Comparison of Power Function to Logit Model
- Conclusions
- Chapter 8. Nonlinear Regression Models
- Introduction
- Regression Models
- Nonlinear Formulation
- Solving Nonlinear Regression Model
- Estimating Parameters
- Nonlinear least squares (Non-OLS)
- Hypothesis Testing
- Evaluate Model Performance
- Sampling Techniques
- Random Sampling
- Sampling With Replacement
- Sampling Without Replacement
- Monte Carlo Simulation
- Bootstrapping Techniques
- Jackknife Sampling Techniques
- Chapter 9. Machine Learning Techniques
- Introduction
- Types of Machine Learning
- Examples
- Classification
- Regression
- Neural Networks
- Chapter 10. Estimating I-Star Market Impact Model Parameters
- Introduction
- I-Star Market Impact Model
- Scientific Method
- Chapter 11. Risk, Volatility, and Factor Models
- Introduction
- Volatility Measures
- Implied Volatility
- Forecasting Stock Volatility
- Historical Data and Covariance
- Factor Models
- Types of Factor Models
- Chapter 12. Volume Forecasting Techniques
- Introduction
- Market Impact Model
- Average Daily Volume
- Observations Over the 19-Year Period: 2000–18
- Observations Over the Most Recent 3-Year Period: 2016–18
- Forecasting Daily Volumes
- Chapter 13. Algorithmic Decision-Making Framework
- Introduction
- Equations
- Algorithmic Decision-Making Framework
- Comparison of Benchmark Prices
- Comparison Across Adaptation Tactics
- Modified Adaptation Tactics
- Chapter 14. Portfolio Algorithms and Trade Schedule Optimization
- Introduction
- Trader's Dilemma
- Transaction Cost Equations
- Optimization Formulation
- Portfolio Optimization Techniques
- Portfolio Adaptation Tactics
- Chapter 15. Advanced Algorithmic Modeling Techniques
- Introduction
- Trading Cost Equations
- Trading Strategy
- Trading Time
- Trading Risk Components
- Trading Cost Models—Reformulated
- Timing Risk Equation
- Comparison of Market Impact Estimates
- Managing Portfolio Risk
- Chapter 16. Decoding and Reverse Engineering Broker Models with Machine Learning Techniques
- Introduction
- Pre-Trade of Pre-Trades
- Portfolio Optimization
- Chapter 17. Portfolio Construction with Transaction Cost Analysis
- Introduction
- Portfolio Optimization and Constraints
- Transaction Costs in Portfolio Optimization
- Portfolio Management Process
- Trading Decision Process
- Unifying the Investment and Trading Theories
- Cost-Adjusted Frontier
- Determining the Appropriate Level of Risk Aversion
- Best Execution Frontier
- Portfolio Construction with Transaction Costs
- Example
- Conclusion
- Chapter 18. Quantitative Analysis with TCA
- Introduction
- Are The Existing Models Useful Enough For Portfolio Construction?
- Pretrade of Pretrades
- How Expensive is it to Trade?
- Backtesting Strategies
- Market Impact Simulation
- Multi-Asset Class Investing
- Multi-Asset Trading Costs
- Market Impact Factor Scores
- Market Impact Factor Score Analysis
- Alpha Capture Program
- Chapter 19. Machine Learning and Trade Schedule Optimization
- Introduction
- Multiperiod Trade Schedule Optimization Problem
- Nonlinear Optimization Convergence
- Machine Learning
- Machine Learning Training Experiment
- Performance Results
- Conclusions
- Chapter 20. TCA Analysis Using MATLAB, Excel, and Python
- Introduction
- Transaction Cost Analysis Functions
- Transaction Cost Model
- MATLAB Functions
- Excel and Python Functions
- TCA Report Examples
- Conclusion
- Chapter 21. Transaction Cost Analysis (TCA) Library
- Introduction
- Transaction Cost Analysis Using the TCA Library
- References
- Index
- Edition: 2
- Published: September 4, 2020
- Imprint: Academic Press
- No. of pages: 612
- Language: English
- Paperback ISBN: 9780128156308
- eBook ISBN: 9780128156315
RK
Robert Kissell
Robert Kissell, Ph.D., is President of Kissell Research Group, a global financial and economic consulting firm specializing in quantitative modeling, statistical analysis, and algorithmic trading. He is also a professor at Molloy College in the School of Business and an adjunct professor at the Gabelli School of Business at Fordham University. He has held several senior leadership positions with prominent bulge bracket investment banks including UBS Securities where he was Executive Director of Execution Strategies and Portfolio Analysis, and at JP Morgan where he was Executive Director and Head of Quantitative Trading Strategies. He was previously at Citigroup/Smith Barney where he was Vice President of Quantitative Research, and at Instinet where he was Director of Trading Research. He began his career as an Economic Consultant at R.J. Rudden Associates specializing in energy, pricing, risk, and optimization. Dr. Kissell has written several books and published dozens of journal articles on Algorithmic Trading, Risk, and Finance. He is a coauthor of the CFA Level III reading titled “Trade Strategy and Execution,” CFA Institute 2019.”
Affiliations and expertise
President, Kissell Research Group; Professor, Molloy College; Adjunct Professor, Fordham UniversityRead Algorithmic Trading Methods on ScienceDirect