Using knowledge mining to fast-track innovative treatments for disease
June 18, 2020
By Ian Evans
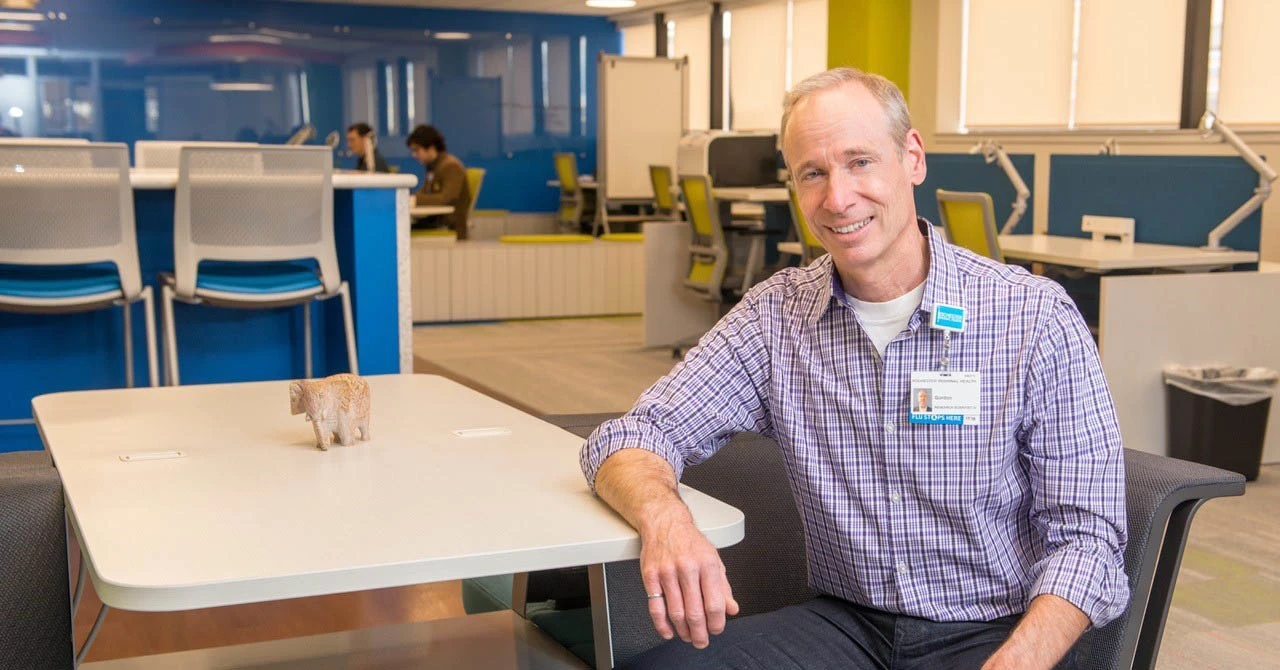
Academic-industry collaboration In Silico Biology creates computer models that tap into how researchers interpret their data
Mathematical models have an important place in science and engineering. They can help scientists explain the dynamic behavior of a system and understand how the components of that system work together. For researchers delving into complex problems, models can help them come to grips with huge amounts of diverse and often incomplete information quickly and accurately.
But the challenge in creating these models varies among disciplines. Can complex systems with gaps in where our knowledge is incomplete or largely qualitative be represented in mathematical models? And can these models allow researchers to predict treatments for rare or new diseases?
Yes, according to collaborative project involving Elsevier’s data scientists and researchers from the University of Rochester. And what’s more, the resulting models can be put to use solving real-world problems in diseases and their treatments.
As Elsevier Data Scientist Dicle Hasdemir opens in new tab/window explained, in some areas of study such as bioinformatics, researchers are faced with incredibly complex systems and limited data with which to model them:
During my academic years in bioinformatics, I had many moments that I felt discouraged because of the complexity of the models we were working on. Biology is not physics. Models have to be built with very sparse data most of the time, and the variance between different organisms, organs or even cells make it hard to tune the models in a satisfactory way. In such conditions, I found it difficult to imagine how these models could be used on real world-problems.
Her doubts were allayed on her first day at Elsevier, when she was introduced to the team behind the academic/industry collaboration In Silico Biology (ISB). That team is creating computer models of biological programs that attempt to connect the puzzle pieces of current knowledge and big data in ways that effectively capture the dynamicity of interaction – and which can ultimately help solve problems in biology, such as how molecular function generates cellular function. Crucial to these models is the idea that when researchers publish a paper, they’re not just sharing data, they’re sharing their interpretation of what that data means. So the models pull together information that’s not just numerical but includes an understanding of the conclusions drawn by the researchers that published the data.
These executable models operationalize informal graphical descriptions (for example, static pathway renditions) with executable processes that add formality, causation and dynamicity to the knowledge base described.
For Dicle, it was a remarkable moment:
Until I met the great interdisciplinary team behind the ISB project in Elsevier, I had no idea how mathematical models in bioinformatics could be applied to real-world problems. When I was handed the first papers regarding this project on my first day at Elsevier, I was as happy as if I’d met an old friend. An extremely clever, dynamic systems biology modeling framework had made its way from academia to industry!
Dicle noted that the task was far from complete. The team needed to develop the modeling process to allow for predictions and turn it into a tool that any researcher could use.
Also on the team was Prof. Gordon Broderick opens in new tab/window, who directs the Clinical Systems Biology research team at Rochester General Hospital in New York.
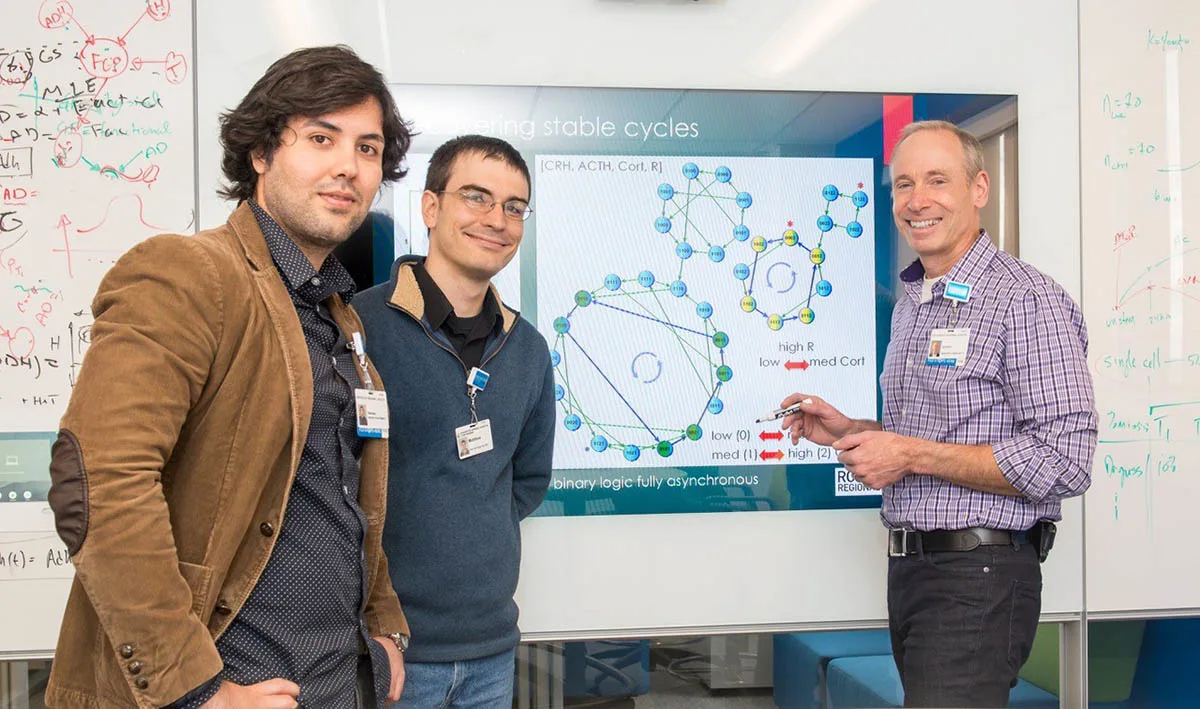
Prof. Gordon Broderick (right) with team members Hooman Sedghamiz and Dr. Matt Morris at the Center for Clinical Systems Biology at Rochester General Hospital in 2017
Gordon has previously worked with Elsevier on ways to use text mining to tackle chronic disease. The ISB collaboration builds on that work, using natural language processing to analyze not just the data but to interpret and make connections based around the conclusions a researcher draws in their data. Gordon explained:
When I publish a paper, I’m not just showing you my data – I’m showing you what I think my data means. In biology, I might be describing the mechanisms that I think the data is telling me about. That’s essentially what we’re capturing – a knowledge model based on how researchers think a complex system works based not on one person but on the total experience of many, many researchers.
In this way, the models the ISB develops draws not just on the numerical data available, but the reasoning and interpretations of the researchers who published articles and drew conclusions based on that data. As Gordon puts it: “We draw on everyone else’s understanding of the data to create our model.”
Someone working on a new research project can then test their own findings against that knowledge model in order to validate them in the broader context of what we think we know. These models build up their accuracy by drawing on the huge amounts of information, understanding and data Elsevier has access to through platforms such as ScienceDirect and Reaxys.
The models are being used to identify the likely success or failure of a new clinical trial, for example, or to identify possibly new treatments for a complex disease.
These mathematical models work as a kind of cause-and-effect map, which Gordon compared to a circuit with interconnecting nodes. Each node is a biological component – maybe a cell population, a soluble mediator or an enzyme – and it interacts with other components in a way that either activates or inhibits them. The team can then propose, from the text interpretations — often mined from tens of thousands of papers and interpreted via natural language processing – the way in which components interact or regulate one another, e.g., how the activation of node A regulates the activation of node D and so forth. Gordon elaborated:
We stitch all of these different interactions together and apply a discrete decisional logic to turn it into a discrete event simulation, so we can model, for example, the sequence of events that has to take place to bring an immune system to rest, or how a virus might affect someone with a specific underlying health condition.
Creating those maps depends on being able to mine and interpret multiple kinds of information and combine the benefits of natural language processing with the essential human component of being able to make inferences, observations and draw conclusions. Gordon explained:
What we do is go out and pull as much information, as many types of information, from as many different sources as we can. We integrate that into a logical network, which then explains how information flows through a given biological system. We model normal physiology, and then we show how an illness can push that physiology into a different state, or make it fail altogether.
For example, using a model of Gulf War illness, Gordon’s team demonstrated that individuals with this complex chronic disease exhibit a steady-state regulatory program that is different from that of healthy individuals. Researchers are then able to model which of the many available treatments or drug combinations propel the diseased state back into a steady healthy state. Gordon and colleagues at the US CDC tested the in silico predicted treatment in Gulf War illness mouse models, curing over 60 percent of the mice. This treatment scenario has now been fast-tracked for testing in Phase 1 clinical trials. Gordon continues to partner with experimental researchers working on other diseases to test his in-silico modeling approach across a broad range of illnesses, with very encouraging results.
Chris Cheadle, PhD
Dr. Chris Cheadle opens in new tab/window, Director of Research for Biology Products at Elsevier, who is also part of the collaboration, explained:
For Gulf War illness alone, Elsevier provided pathway knowledge extracted from over 20,000 research papers. This saved significant research time and provided information that might not be discovered by manual review alone. Analytics also reduces the need and costs for computing power as researchers can use machine understanding (semantic) approaches to summarize and extract critical information. This reduces the data error for the extrapolation of the typically small data sets in rare disease fields and for larger data sets as well.
The computer modeling approach provides more and new treatment options and is now part of Entellect, Elsevier’s new biology platform. It helps reduce unnecessary and overlapping experiments as well as helping researchers understand which approaches are likely to fail. That can make work in drug discovery much more efficient, more expeditious and less resource-intensive.
For example, in a rapid response to the coronavirus epidemic, the team currently modeling how individuals with chronic obstructive pulmonary disease (COPD) respond to respiratory infection has leveraged this ongoing research to create, in a matter of weeks, a prototype mechanistic model of end-stage cytokine storm in COVID patients. Gordon explained:
We’ve taken our COPD model and thought about what we need to add to that. In this instance, we’ve combined our understanding of immune hyper-responsiveness with relatively sparse data and information related to SARS. With that, we can explain some of the clinical observations and some of the outcomes related to coronavirus.
As Dicle noted when she first encountered the work being done by In Silico Biology, the key is now to develop this work into a platform that researchers can use to tackle their specific challenges. Gordon said the partnership with Elsevier is helping drive that process to a level – and with a momentum –previously unimaginable for a purely academic team:
Elsevier has helped us join those dots. We have experts from academia and a number of pharmaceutical companies sitting at the table. And we're all exchanging ideas as to how we approach integration across all mixed platforms, so that these knowledge graphs can plug into the tools that each company or lab is using.
From there we will look at how use this technology for the planning of clinical trials and target identification. It’s an exciting journey!
Contributor

IE