Research data
Making data effective
At Elsevier we believe there are 10 aspects of highly effective data and which can function as a roadmap for the development of better data management processes and systems throughout the data lifecycle. These are detailed below:

Stored: The first step in the hierarchy of research data needs is that data that have been acquired need to be stored.
Preserved: Once research data is stored, it then needs to be preserved in a format-independent manner or risk data obsolescence.
Accessible: Even when data is stored and preserved, this does not necessarily mean it is automatically accessible. Both researchers and machines may want to access the data, for example, for meta-analyses or other kinds of re-use.
Discoverable: Even if data are stored, preserved and in principle accessible, this is not very worthwhile if the data cannot be discovered by others.
Citable: One of the barriers to data sharing has been that it requires extra work from researchers for little reward. Data citations have the potential to change that because they can be easily incorporated in the current reward system based on article citations.
Comprehensible: To enable data to be reused, it needs to be clear which units of measurements were used, how the data was collected and which abbreviations and parameters are used. Data provenance is crucial for comprehension.
Reviewed: While it is very common for research articles to be peer reviewed, this is still quite uncommon for research data. However, it is an important step when it comes to quality control and trustworthiness of data.
Reproducible: Reproducibility of research results is a big concern for science. Irreproducibility often originates from missing elements to research data, which are needed in order to achieve the same research results. For example resources (e.g., antibodies, model organisms, and software) reported in the biomedical literature often lack sufficient detail to enable reproducibility or reuse.
Reusable: The key benefit for the wider research community of having research data being shared is the ability to reuse this data. Only when research data is sufficiently trustworthy and reproducible will other researchers re-use the data.
Integrated: We believe that it is important to integrate these nine aspects of “highly effective research data.” For instance, data should be preserved so that it can be reused. To be citable, it needs to be accessible. But also, in building systems for data reuse or data citation, the practices of current systems for storing and sharing data need to be taken into account.
These nine layers and 10th integration step are intended as a guiding principle by which research data management practices can be ordered and checked, rather than as a prescription for perfect performance.
Elsevier contribution to the research data community
Co-founder Co-author FAIR Data principles Implementation data citations principles | |
Co-founder | |
Active member | |
Scholix | Co-founder |
Supporting Brussels open data declaration | |
Active member |
Elsevier solutions for research data
Text and data mining
All Elsevier journals and books enable text and data mining (TDM). Find out how you can work more efficiently today.
Data citation
All Elsevier journals facilitate the citation in a consistent and clear manner in the scientific literature.
Related information
Read moreOpen data
Read moreAlthough the benefits of open research data are well known, in practice, confusion remains within the researcher community around when and how to share research data.
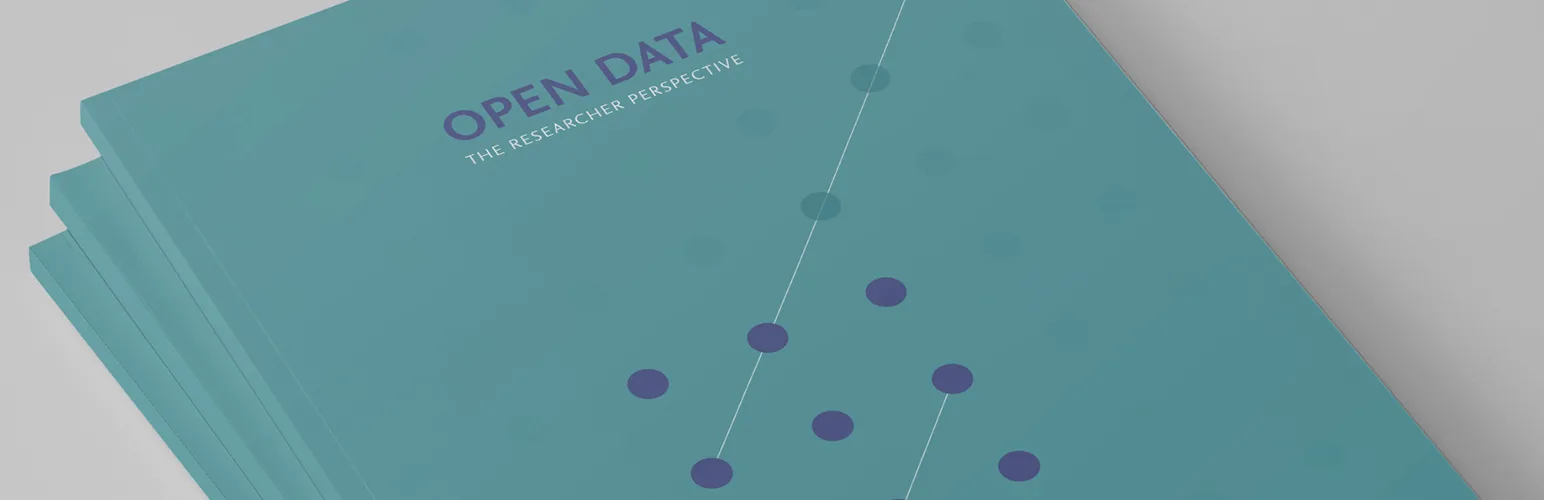