How data sharing is accelerating railway safety research
11 janvier 2021
Par Libby Plummer
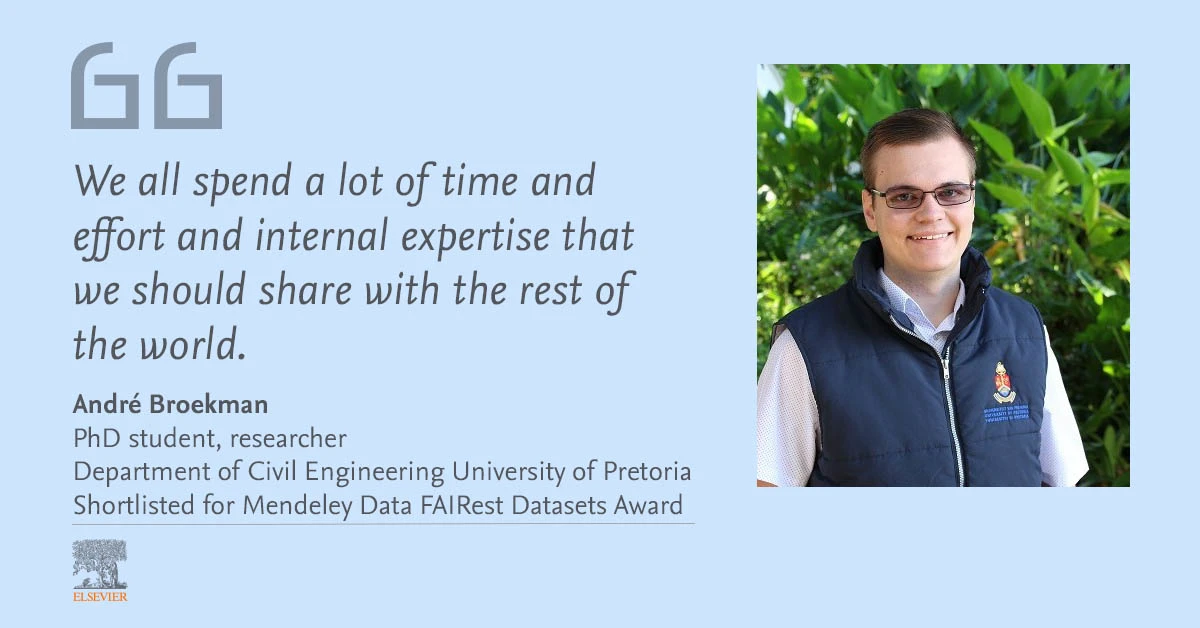
Civil Engineering researcher André Broekman is developing an AI-based tool to create photorealistic virtual environments in the lab
Pictured above: André Broekman is a PhD student in the Department of Civil Engineering at the University of Pretoria. His dataset was shortlisted for the Mendeley Data FAIRest Datasets Award.
The sharing of data is a vital tool for many researchers around the world, and for André Broekman S’ouvre dans une nouvelle fenêtre, a PhD student at the University of Pretoria S’ouvre dans une nouvelle fenêtre, it has proven to be essential. In the Department of Civil Engineering S’ouvre dans une nouvelle fenêtre, André was able to use an existing neural network shared by a researcher from another university to boost his own research.
He used a neural network developed by Associate Professor Dr Yao Yao of the Hong Kong Polytechnic University S’ouvre dans une nouvelle fenêtre.
"As there was already this framework in place that I could build on, this allowed me to accelerate my own research projects," André explained. "When you can spend less time developing your own specific dataset, this is very time and cost effective. And after I've added my own unique characteristics and properties to the research, this can then be shared back into the community, where somebody else might benefit from it."
Many of the projects in his department are driven directly by industry needs, including André’s latest research, which aims to incorporate AI technologies into railway condition monitoring:
If you can't measure it, you can't manage it. Not only can this improve safety on the rails, it can also help rail operators to run more efficiently.
He said the university has close ties to industry, so the research is being undertaken as part of a collaboration between Chair in Railway Engineering Prof Hannes Gräbe S’ouvre dans une nouvelle fenêtre and Australian engineering firm 4Tel S’ouvre dans une nouvelle fenêtre, which builds digital rail network management systems.
André's latest dataset S’ouvre dans une nouvelle fenêtre has been recognized by the Mendeley Data FAIRest Datasets Award S’ouvre dans une nouvelle fenêtres and described by the judges as “a Perfectly Accurate, Synthetic dataset for Multi-View Stereopsis (PASMVS).” It comprises 400 scenes and 18,000 model renderings generated using Blender S’ouvre dans une nouvelle fenêtre open source software. The idea is that 2D images can be used to create 3D photorealistic virtual environments.
Using photographs to acquire data for 3D modeling
The 3D renderings in this particular dataset are limited to a small selection of objects, including a teapot and the Stanford bunny — two well-known test models in the computer graphics community. The idea is that eventually this technique can be used to create incredibly precise 3D renderings of real-world railways in the lab. This would enable technicians to assess their condition in a cost-effective way.
In the image: Visual collage of 8 scenes illustrating the variability introduced by using different models, illumination, material properties and camera focal lengths. (Source: André Broekman and Petrus J. Gräbe: PASMVS: a dataset for multi-view stereopsis training and reconstruction applications S’ouvre dans une nouvelle fenêtre via Mendeley Data)
"This dataset is actually just a small stepping stone,” André explained. “It serves as a way to develop a pipeline to create these datasets for rail condition monitoring in future. We've already produced another dataset of the recreated railway environment as the final stepping stone before we move on to field trials."
By mounting cameras onto a dedicated inspection vehicle, or even a freight or passenger train, the researchers would be able to gather the necessary images to recreate accurate 3D readings of the rails and all the components that surround them back in the lab. Field trials will help them figure out exactly what kind of cameras are needed and where to place them. Because the actual rails on railway lines tend to be reflective, the ability to accurately recreate reflective surfaces was a key focus when using Blender's AI-powered 3D modeling.
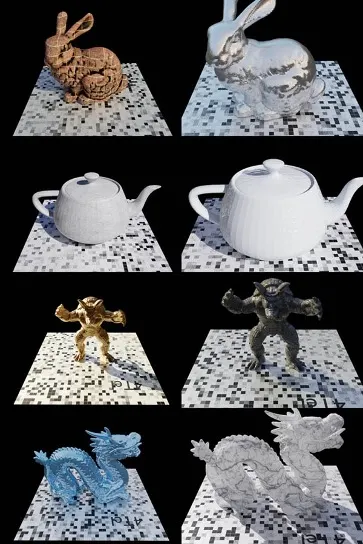
Using photographs is a relative cheap and easy way to acquire data for 3D modeling, and for some applications, the dataset might require just a few images taken with a smartphone. Applying research to rail transport and “Civiltronics”
While the eventual goal of André's dataset is to develop an AI-based rail monitoring system for railway infrastructure, the technique could eventually be used in other ways as well. André is already looking at using the application to monitor the condition of roads. Moving more towards agriculture, the university also has a synthetic dataset project running that is designed to count bees using a video camera and a similar workflow that can train a neural network to do the job.
For André's dataset, the hope is that field trials can eventually start in spring 2021. The university has a 30-meter section of railway track on campus, though access to a full railway line where the product can be validated is an essential part of the plan.
André was initially inspired to pursue the rail-related pathway of civil engineering by the development of the landmark Gautrain train system S’ouvre dans une nouvelle fenêtre, which was opened just in time for the 2010 FIFA World Cup S’ouvre dans une nouvelle fenêtre in South Africa. The ground-breaking rapid railway runs between Pretoria and Johannesburg; it was temporarily shut down in 2020 for the first time in a decade due to the pandemic. "That's where my first interest came from, but I've always been really interested in computers and electronics,” André said. “When I started my master's, we were also incorporating 3D printing software and programming, which I really enjoyed.”
Combining new technologies such as AI, IoT and computer vision with a more traditional systems-based approach to civil engineering has enabled the university to add a great deal of functionality and understanding to its latest projects. The head of the Civil Engineering Department, Prof. Wynand Steyn, came up with the term “Civiltronics” to describe this new approach. The department recently opened a new state-of-the-art Engineering 4.0 campus S’ouvre dans une nouvelle fenêtre, which enabled André to research and create his latest dataset.
The Mendeley Data FAIRest Datasets Award
André's dataset was shortlisted for the Mendeley Data FAIRest Datasets Award, which recognizes researchers who make their data available for the research community in a way that exemplifies the FAIR Data Principles S’ouvre dans une nouvelle fenêtre– Findable, Accessible, Interoperable, Reusable. The dataset was applauded for a number of reasons, not least the provision of clear steps to reproduce the data. What's more, the data was clearly catalogued and stored in sub folders, with additional links to Blender and GitHub, making the dataset easily available and reproducible for all.
As a "one-man show" in this particular research project, André was surprised but pleased to be shortlisted for the award:
It was a nice feeling as the dataset is part of my first journal publication. It really validated the good research that we're doing and made me feel that it could really make an impact. It also helps to give our department a bit more visibility.
André’s suggestions for sharing research data
André is keen to highlight the importance of shared data and the boost to his research that he was able to get by using Dr Yao's neural network framework. While data sharing is still in its early days in the civil engineering field, the university is actively encouraging a culture of open data both on campus and on a wider scale. André explained:
We're actively implementing easy-to-use data repository services such as Mendeley Data S’ouvre dans une nouvelle fenêtre to make our datasets readily available and encouraging our department staff to make use of such services.
André said Mendeley Data S’ouvre dans une nouvelle fenêtre offers a number of benefits, in particular the ability to give a detailed description and reproducibility steps, which is not always included in the journal article. He also highlighted the platform's ample storage space, reliability and longevity, which is not always offered by non-persistent data sources such as Google Drive. The ability to easily download supporting files and images related to the dataset is also a major plus point. The only addition that André would like to see is a little more interactivity:
It would be great if you could have a little viewer running so you can look at objects in three dimensions and zoom in and out. Improving the viewing functionality would just add so much more in terms of how you can view and move around 3D objects in a virtual space.
André’s work is a clear example of the benefits of sharing research and datasets and highlights how a collaborative mindset can push research forward.
If you publish your datasets and tell other students and researchers about it, then we can all get on that train, so to speak, and it's beneficial for everyone. We all spend a lot of time and effort and internal expertise that we should share with the rest of the world.
Contributeur

LP