For Mendeley Data winner, sharing FAIR data helps researchers learn from each other
2020年9月4日
Ian Evans別
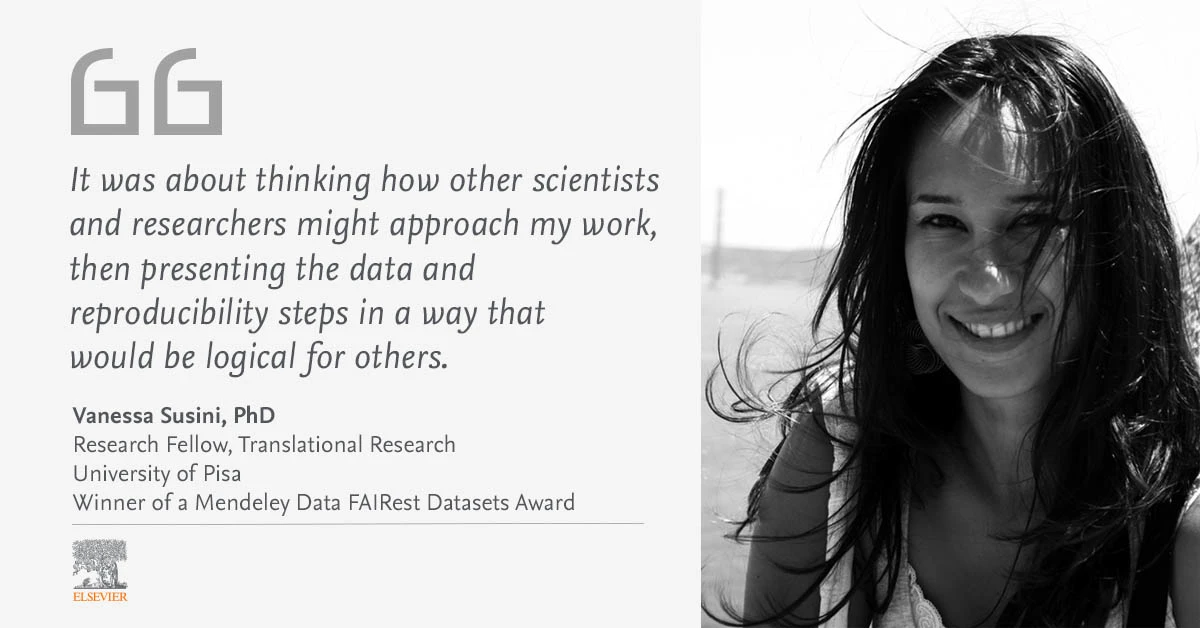
A research fellow talks about how to create meaningful datasets – and why sharing them benefits all involved
Pictured above: Dr. Vanessa Susini, a Research Fellow in the Department of Translational Research and New Technologies in Medicine and Surgery at the University of Pisa, is a recent winner of the Mendeley Data FAIRest Datasets Award.
For Dr. Vanessa Susini 新しいタブ/ウィンドウで開く, one of the most significant benefits of sharing data is the way it helps people in the research community learn from each other.
Vanessa, a Research Fellow in the Department of Translational Research and New Technologies in Medicine and Surgery at the University of Pisa 新しいタブ/ウィンドウで開く, is a recent winner of the Mendeley Data FAIRest Datasets Award 新しいタブ/ウィンドウで開く. The award recognizes researchers or research groups that make their research data available for additional research and do so in a way that exemplifies the FAIR Data Principles 新しいタブ/ウィンドウで開く– Findable, Accessible, Interoperable, Reusable.
As Wouter Haak, VP of Data Management Solutions at Elsevier, noted:
Sharing data in a FAIR way is often seen as an extra burden to researchers, who already burdened with many other tasks. Indeed, with the dataset from Vanessa Susini and her team, they had to do extra work to make it FAIR.
So why make this extra effort?
For Vanessa, creating datasets that adhere to those principles is a way of ensuring that she and her peers continue to develop and grow as researchers:
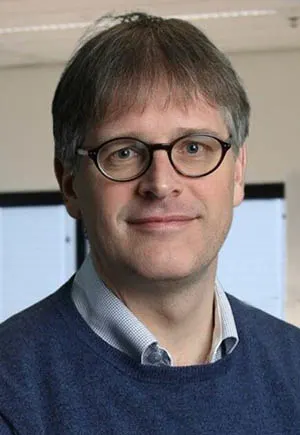
Wouter Haak
What’s most important to me is capturing inputs accurately so that other researchers have what they need to recreate our work at their lab bench. That way, you can share it with other people in your research community, they can get in touch with you, and you can brainstorm together. You can find new way to think about the data. You learn off each other.
Vanessa’s research focuses on the development of immunoassays and, in particular, examines the effect of the chemical reduction on antibodies used in immunoassays based on oriented antibodies. Immunoassays 新しいタブ/ウィンドウで開く are a bioanalytical method that measure the presence or concentration of the substance being analyzed – with substances ranging from small molecules to macromolecules in a solution. They’re used in a wide variety of settings, including environmental monitoring, security and food testing, and Vanessa’s work plays a role in developing highly sensitive immunoassays that are low-cost and easy to use.
“Our contribution is not so much about developing a particular high sensitive immunoassay for a certain analyte, but it’s about understanding why antibody reduction enhances the sensitivity of these tests,” she explained. “So it’s broader, and because of that, it’s vitally important that the data is captured well and is interoperable between labs.”
To that end, Vanessa and her team paid particular attention to input methods and equipment that you would commonly find in other laboratories. They also included steps on reproducing the research, and shared those steps and the accompanying dataset on Mendeley Data 新しいタブ/ウィンドウで開く.
In a sense, it was just about thinking how other scientists and other researchers might approach my work, and then presenting the data and reproducibility steps in a way that would be logical for others.
Indeed, that is her key piece of advice for others looking to create datasets that are meaningful for other teams:
You need to think about the fact other people are reading these words and start from there. What do they need, how will they find it, what will make sense to them? Think about the parameters they will need, how they might search for this information and input data accurately right down to the kind of equipment you used during the experiment.
From Wouter’s experience, that attitude of taking the extra steps to ensure other researchers have good data to build on is becoming more and more prevalent:
I think Vanessa and her group, like increasingly many other research groups, have recognized that research outcomes spread more quickly if the data is well described and made reproducible. Making your data FAIR is a network benefit: it benefits those who come after you, and by properly publishing the data, the article and/or data will be used and cited so much more. So your findings get multiplied more quickly.
All researchers like to build on prior research in a way that can get them started more quickly, so do as Vanessa says: attract more collaborations by making your data FAIR!
Indeed, as Vanessa describes it, the kind of open science approach that data sharing represents is a particularly effective way of breaking down boundaries between disciplines and driving a more collaborative future:
There can be a lot of compartmentalization of knowledge in research. It’s not always the case that people reach across disciplines. But the kind of work I do is relevant to physics, to chemistry, to biology and more, so sharing the datasets and hearing back from others becomes a way to break down those boundaries and learn something from each other.
View Vanessa’s dataset and those of other winners
We are interviewing researchers who made their research data available in a way that exemplifies FAIR data principles — Findable, Accessible, Interoperable, Reusable. The researchers are chosen by the Elsevier RDM Advisory Board and Elsevier Data Champions. You can view and download their datasets in the Mendeley Data Repository 新しいタブ/ウィンドウで開く.
More RDM resources
貢献者

IE