Key components of developing AI literacy at your institution
2024年1月16日 | 7 分経過
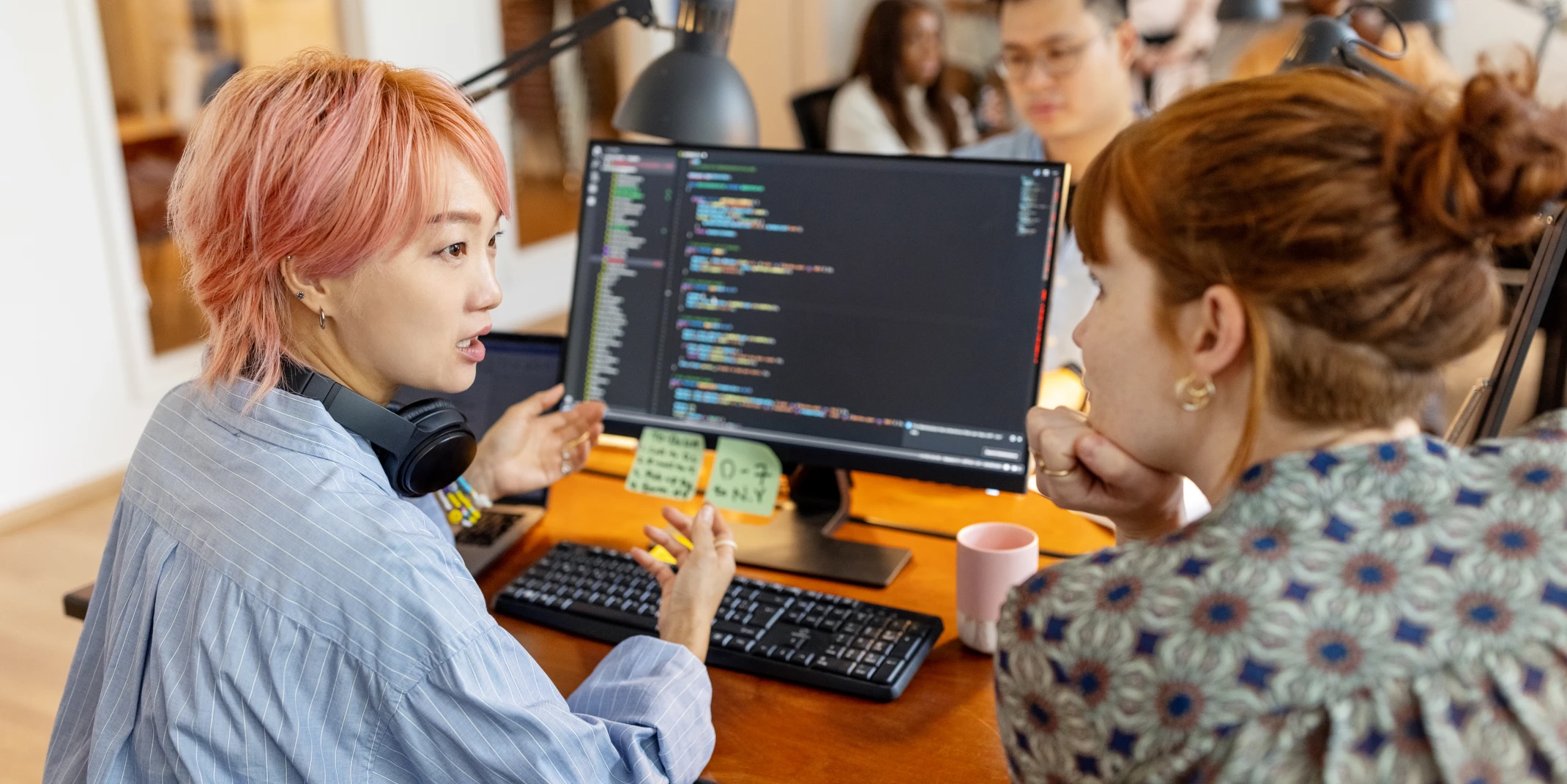
Librarians are indispensable in shaping a future where AI-generated information is not only abundant but also credible, reliable, and accessible to all.
In an era where information is abundantly generated and disseminated, the rise of Artificial Intelligence (AI) has significantly transformed the way we access and interact with information. However, as the dependency on AI-generated content grows, the critical issue of user trust in the authenticity and credibility of AI-generated information emerges as a pressing concern. AI-generated content often presents users with a complex web of information that can be challenging to navigate.
Additionally, the opacity of AI algorithms and the lack of transparency in the decision-making processes can create skepticism and doubt among users. How reliable is the information produced by AI? What are the frameworks evaluating the credibility of AI technologies?
In this context, librarians, renowned for their expertise in information curation, verification, and dissemination, are uniquely positioned to bridge the gap between users and AI-generated information. Librarians can serve as interpreters and mediators, facilitating a deeper understanding of the intricacies of AI technologies and their impact on information retrieval. Librarians can also enhance user trust by supporting AI literacy and advocating for transparency in AI systems.
Educating Users: AI literacy on campus
To begin evaluating AI, users must first understand it or become AI literate. AI literacy, consists of knowing, understanding, using, and evaluating AI, as well as considering the ethical issues (Ng, et al, 2021). AI literate individuals also understand fundamental AI concepts like machine learning, natural language processing, and neural networks. To equip students, researchers, and faculty members with the necessary skills to navigate this complex landscape, libraries need to prioritize developing training resources that enable individuals to scrutinize information concerning AI applications. When library users grasp the capabilities and constraints of AI, they can properly assess AI-driven tools and resources.
AI literacy concepts can be taught in many of the traditional literacy avenues, such as information literacy, media literacy, and digital literacy. Remember, you don’t need to be a computer expert to create or attend AI literacy workshops. The crux of AI literacy lies not in technical expertise but in fostering critical thinking.
Alongside a fundamental grasp of AI concepts, AI literacy also involves the capability to critically evaluate AI technologies. Using a structured approach or specific questioning strategies for the evaluation can play a pivotal role in facilitating more meaningful discussions, ultimately leading to an improved understanding and critical evaluation. Below are some techniques to begin this process, along with structured questions tailored to guide non-technical individuals.

The ROBOT Test
One particularly useful resource is known as the ROBOT Test (Wheatley and Hervieux, 2020). It was developed by two librarians at McGill University, Amanda Wheatley and Sandy Hervieux to offer a structured framework for individuals new to AI to evaluate new information related to AI technology.
ROBOT, an acronym for reliability, objective, bias, ownership, and type, delineates key criteria for assessing information about AI tools. Each word in the acronym comprises a set of questions that guide users through a comprehensive evaluation process. For instance, to discern the type of an AI tool, users can pose questions such as:
What specific category of AI does it belong to?
Is the technology more of a concept or something that's being used practically?
What type of information system is it dependent on?
Does it require human involvement?
A complete outline of the ROBOT test and the questions it prompts the user to ask can be found on the McGill Library AI Literacy website 新しいタブ/ウィンドウで開く.
Introducing the GenAI Literacy program for librarians!
GenAI comes with a lot of questions. And we can help. Over three self-paced professional development courses, you will:
Develop fundamental AI literacy skills
Critically assess the benefits and limitations of AI
Responsibly approach and evaluate AI tools
Earning your certification is the first step in empowering yourself and your library with the skills and knowledge to navigate GenAI.

Evaluation and developing explainability
AI literacy also involves being able to evaluate and explain these technologies. New users are likely to view an AI system like a “black box” where information goes into the system and an answer comes out without an understanding of how it arrived at the answer. Being able to have a better explanation allows users to see into the black box and consider how the inputs are used to create the outputs. This is an essential step in building user trust. Librarians can and should advocate for increased transparency within AI systems and encourage developers and tech companies to divulge the inner workings of AI-generated content.

However, the intricate nature of AI systems often poses a challenge during dialogues between developers and end-users. Non-technical individuals, in particular, might find it challenging to effectively articulate their inquiries, even when provided with opportunities to seek explanations. After all, you do not know what you do not know. Not knowing the topic well, it is hard to predict the appropriate questions. This highlights the necessity for users to become familiar with basic AI concepts before evaluating the more complex AI systems.
In the next two sections, we lay out types of explanations and key components of explainability with some exemplary questions to guide users in their exploration and evaluation of AI systems.
Types of explanations of AI systems
An explanation starts with understanding the capabilities of the machine learning (ML) systems (Cabitza et al, 2023). This includes acknowledging what these systems can or cannot offer users and recognizing areas where human decision makers still need to provide interpretation and judgment.
Users can then start to look at the functionality of the AI system, its outputs and more. In a recent paper, Cabitza et al. presented a comprehensive overview identifying various types of explanations of AI systems and the criteria for evaluating their quality (2023). They proposed the following key questions in seeking explanations for AI systems: “
Computational explanation: How does the algorithm A produce any output O?
Mechanistic explanation: Why does the algorithm A have produced the output O?
Justificatory explanation: Why is the output O right?
Causal explanation: What is the physical phenomenon that causes O?
Informative explanation: What does the output O mean?
Cautionary explanation: What is the uncertainty behind the output O?”
By walking through these different areas of explanation, users can start to demystify the “black box” of an AI system and gain confidence in using AI systems as tools to navigate their research or coursework.
Key components of explainability
What counts as a good explanation for AI? To aid in establishing the criteria for explaining AI systems, Balasubramaniam and colleagues (2023) proposed a framework outlining the key components of explainability. This model serves as a guide for practitioners, helping them pinpoint the specific requirements for explainability by addressing crucial inquiries:
Addressees (Ask yourself to whom the explainer tries to explain?)
Aspects (What to explain?)
Context (What are the contextual situations requiring explanation?)
Explainer (Who explains?)
Encourage users to seek answers to each of these components when asking questions to explain AI systems.
Elsevier acknowledges that transparency and explainability has become emerging quality requirements of AI systems. Within its Explainable AI system, this comprehensive video below explains the technology behind Scopus AI.
Watch the video below ↓
Tip: Try to apply the evaluation frameworks and key questions above in the article when watching the video.
The Technology behind Scopus AI

Watch now
|
The Technology behind Scopus AI
Librarians can build trust in AI technologies
By actively engaging in AI literacy initiatives, librarians can play an instrumental role in empowering users to make informed decisions about the credibility of AI-generated information. Through their expertise in information literacy and their commitment to promoting transparent and ethical information practices, librarians can instil a sense of trust and confidence in the reliability of AI technologies.
In the pursuit of fostering user trust in AI technologies, collaboration between librarians, AI developers, and policymakers is essential. Establishing a multidisciplinary approach that prioritizes transparency, education, and ethical considerations can pave the way for a more trustworthy and accountable AI ecosystem. Librarians are indispensable in shaping a future where AI-generated information is not only abundant but also credible, reliable, and accessible to all.
Dive deeper into AI topics for librarians:
The role of AI in library services looks at making AI more accessible at your institution, including recommendations for supporting researchers and students.
Addressing bias in AI-fueled knowledge systems discusses the way librarians and researchers are working together to combat the biases in AI models.
References
Abedin, B., Meske, C., Rabhi, F., & Klier, M. (2023). Introduction to the minitrack on explainable artificial intelligence (XAI).
Balasubramaniam, N., Kauppinen, M., Rannisto, A., Hiekkanen, K., & Kujala, S. (2023). Transparency and explainability of AI systems: From ethical guidelines to requirements. Information and Software Technology, 159, 107197.
Borrego-Díaz, J., & Galán-Páez, J. (2022). Explainable Artificial Intelligence in Data Science: From Foundational Issues Towards Socio-technical Considerations. Minds and Machines, 32(3), 485-531.
Buijsman, S. (2022). Defining explanation and explanatory depth in XAI. Minds and Machines, 32(3), 563-584.
Cabitza, F., Campagner, A., Malgieri, G., Natali, C., Schneeberger, D., Stoeger, K., & Holzinger, A. (2023). Quod erat demonstrandum?-Towards a typology of the concept of explanation for the design of explainable AI. Expert Systems with Applications, 213, 118888.
Kangra, K., & Singh, J. (2022). Explainable Artificial Intelligence: Concepts and Current Progression. In Explainable Edge AI: A Futuristic Computing Perspective (pp. 1-17). Cham: Springer International Publishing.
Kasinidou, M. (2023, June). AI Literacy for All: A Participatory Approach. In Proceedings of the 2023 Conference on Innovation and Technology in Computer Science Education V. 2 (pp. 607-608). https://doi.org/10.1145/3587103.3594135 新しいタブ/ウィンドウで開く
Kong, S. C., Cheung, W. M. Y., & Zhang, G. (2022). Evaluating artificial intelligence literacy courses for fostering conceptual learning, literacy and empowerment in university students: Refocusing to conceptual building. Computers in Human Behavior Reports, 7, 100223. https://doi.org/10.1016/j.chbr.2022.100223 新しいタブ/ウィンドウで開く
Nagahisarchoghaei, M., Nur, N., Cummins, L., Nur, N., Karimi, M. M., Nandanwar, S., ... & Rahimi, S. (2023). An empirical survey on explainable ai technologies: Recent trends, use-cases, and categories from technical and application perspectives. Electronics, 12(5), 1092.
Ng, D. T. K., Leung, J. K. L., Chu, K. W. S., & Qiao, M. S. (2021). AI literacy: Definition, teaching, evaluation and ethical issues. Proceedings of the Association for Information Science and Technology, 58(1), 504-509. https://doi.org/10.1002/pra2.487 新しいタブ/ウィンドウで開く
Hervieux, S. & Wheatley, A. (2020). The ROBOT test [Evaluation tool]. The LibrAIry. https://thelibrairy.wordpress.com/2020/03/11/the-robot-test 新しいタブ/ウィンドウで開く
Quakulinski, L., Koumpis, A., & Beyan, O. D. (2023). Transparency in Medical Artificial Intelligence Systems. International Journal of Semantic Computing.