The miracle of the commons and the democratization of science
5. Mai 2023
Von Daphne Koller, PhD
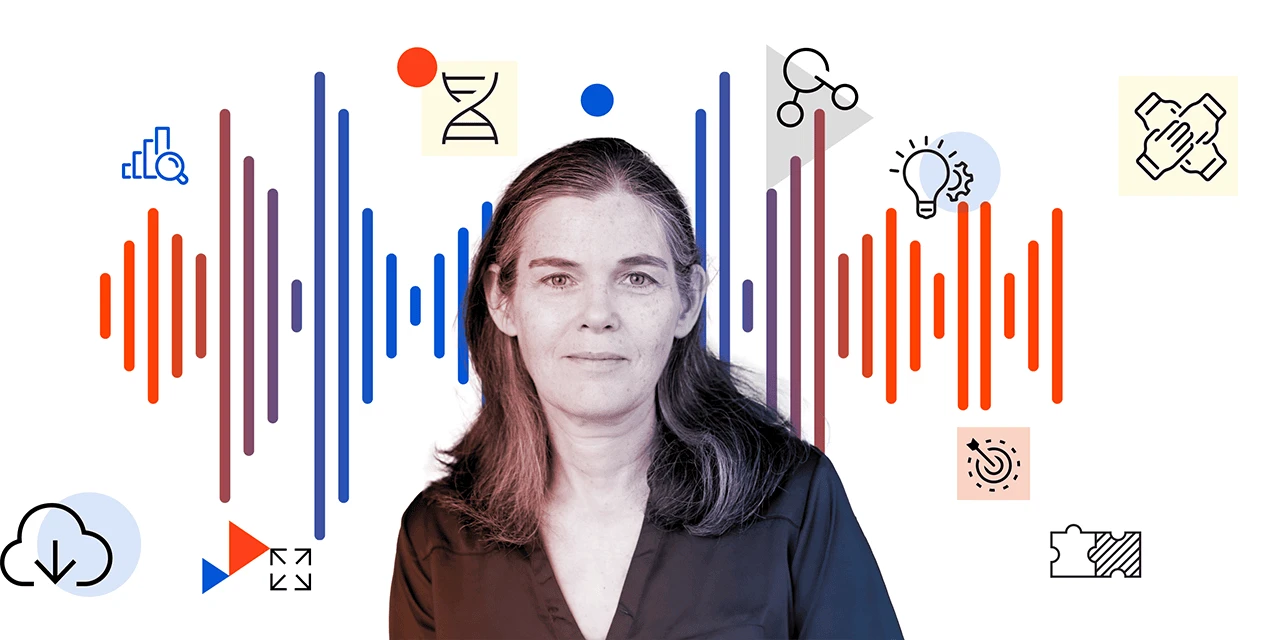
As technology and data allow more people to contribute to science, we need to ensure we are creating knowledge and tools that are egalitarian, open and universal
In the early days of scientific inquiry, it was quite common for a “citizen scientist” — one without an affiliation to an academic institution and sometimes no formal scientific training Wird in neuem Tab/Fenster geöffnet — to contribute significantly to scientific discovery. Noteworthy examples include Leonardo da Vinci Wird in neuem Tab/Fenster geöffnet, Antonie van Leeuwenhoek Wird in neuem Tab/Fenster geöffnet, Benjamin Franklin Wird in neuem Tab/Fenster geöffnet, Mary Somerville Wird in neuem Tab/Fenster geöffnet, Mary Anning Wird in neuem Tab/Fenster geöffnet, Charles Darwin Wird in neuem Tab/Fenster geöffnet, Gregor Mendel Wird in neuem Tab/Fenster geöffnet and Thomas Edison Wird in neuem Tab/Fenster geöffnet. Even Marie Curie Wird in neuem Tab/Fenster geöffnet, although on staff at the École Normale Supérieure Wird in neuem Tab/Fenster geöffnet, conducted much of her work without official support, in a leaky, uninsulated shed.
The contributions made by these scientists were transformative and even led to the birth of entire scientific disciplines.
Over time, however, it became increasingly difficult for “scientific outsiders” to make such contributions. New findings were largely built on an earlier body of knowledge, and accessing that knowledge was hard — not only requiring years of learning but having much of that learning locked within costly books and journals available only to a few. Moreover, much of scientific experimentation relied on highly specialized and expensive equipment and reagents. In addition, high-impact scientific publication was largely disseminated via selective publication venues whose reviewers were insiders who preferentially accepted papers from other insiders.
This post is from the Not Alone newsletter, a monthly publication that showcases new perspectives on global issues directly from research and academic leaders.
Open science reemerges
An exception to this trend has been emerging in the field of machine learning (ML), where the past decade has seen an explosion of work by many diverse researchers. For example, an analysis of the NeurIPS conference Wird in neuem Tab/Fenster geöffnet (among the premier in ML) shows that in 2013, papers came from about 200 institutions, compared to about 1,300 in 2020. This growth was enabled by the ready availability of three important resources:
Extensive open-source code (starting with OpenCV Wird in neuem Tab/Fenster geöffnet and then TensorFlow Wird in neuem Tab/Fenster geöffnet and PyTorch Wird in neuem Tab/Fenster geöffnet) has hugely reduced both the time and expertise needed for writing ML code.
Cloud computing has eliminated the need to obtain access to expensive compute infrastructure.
Large, high-quality data sets: ImageNet Wird in neuem Tab/Fenster geöffnet, published in 2012, was one of the most significant catalysts of the current AI revolution; and the multi-petabyte, freely available web crawling data provided by the nonprofit CommonCrawl Wird in neuem Tab/Fenster geöffnet is one of the key data sets for training the current generative AI models.
All of these reduced the barrier to entry for newcomers in the field and allowed people from all over the world to both utilize the technology and contribute new insights.
Reading the above description, with its emphasis on compute infrastructure and data, one might believe that this type of impact is specific to the ML field. I argue that high-quality, accessible data can have a huge impact in other disciplines. The biomedical sciences have a rich history of such projects, starting with the Human Genome Project Wird in neuem Tab/Fenster geöffnet (HGP), a community-driven effort that brought together more than 2,000 researchers from around the world. The economic, scientific and medical impacts of this effort are enormous. As one example, early drugs were found largely by serendipity, and their molecular and protein targets were often unknown; post-HGP, the targets are known Wird in neuem Tab/Fenster geöffnet for almost all new drugs. From an economic perspective, a 2011 report Wird in neuem Tab/Fenster geöffnet (updated Wird in neuem Tab/Fenster geöffnet in 2013) shows that by 2010, the HGP — which cost $3.8 billion in investment — gave rise (directly and indirectly) to an economic (output) impact of $965 billion, personal income exceeding $293 billion, and 4.3 million job years of employment. The ongoing Moore’s law for genome sequencing Wird in neuem Tab/Fenster geöffnet owes its origins to technology created as part of the HGP.
The HGP was the model for multiple other “open science” projects in biomedical science, many of which have had huge impact in their own right. For example, The Cancer Genome Atlas Wird in neuem Tab/Fenster geöffnet (TCGA) has produced Wird in neuem Tab/Fenster geöffnet over 2.5 petabytes of data, spanning 11,000 patients and 33 tumor types. By providing a rich “molecular catalog” of cancer, it has enabled Wird in neuem Tab/Fenster geöffnet scientists from all over the world to study cancer processes and derive important insights. For instance, we now know that cancer can be driven by a range of both genetic and epigenetic changes and that cancers of different tissues are often actually driven by the same alterations and can be biologically more similar to each other than to other tumors of the same tissue of origin. This insight has been the underpinning of the field of precision oncology.
In a more recent example, the UK Biobank Wird in neuem Tab/Fenster geöffnet (UKB) contains deep, high-quality data on 500,000 anonymized individuals, spanning genetics, multiple phenotypic modalities (including imaging and ‘omics) and longitudinal health follow-up. Due to its open nature, the Biobank was able to attract $480 million in external investment over the past five years (supplementing $40 million in core funding). The richness of the cohort has therefore increased considerably over time, translating into increased impact: in 2015, fewer than 100 publications cited the cohort; in 2022, that number was over 2,200. The UKB played a critical role in understanding the population impact of COVID-19, including long-term consequences on brain Wird in neuem Tab/Fenster geöffnet and cardiovascular Wird in neuem Tab/Fenster geöffnet health. A key factor in the explosive utilization of the UKB resource is its ease of access while preserving privacy. Similar data sets of high potential value (such as deCODE Wird in neuem Tab/Fenster geöffnet or the Million Veterans Program Wird in neuem Tab/Fenster geöffnet) have had considerably less impact because they have been locked away and out of reach for most researchers.
Enabling open science
Recent developments have the potential to enable a sea change whereby researchers from all over the world and diverse backgrounds can contribute to our scientific knowledge. However, we must be vigilant and proactive in ensuring that these tools help decrease rather than increase the gap between the haves and the have-nots, in which capabilities are available disproportionately to certain groups or deployed disproportionately to certain problem domains versus others. We need to make sure we create knowledge and tools that are egalitarian, open and universal.
One important trend is the ongoing shift towards open access knowledge, including both open courseware and the increasing adoption (sometimes even mandated Wird in neuem Tab/Fenster geöffnet) of early and open access publishing, whether in preprint servers or open access journals. This is particularly critical for researchers who aren’t affiliated with wealthy academic institutions and industries.
Another, equally important transformation is the democratization of programming — a critical 21st-century skill that has been consistently challenging to teach to a broader audience. Higher-level programming languages were the first step in making programming more accessible, followed by increasingly large code libraries. But these are nothing compared to the phase transition that is now upon us with the remarkable advent of generative AI models that are able to produce working code Wird in neuem Tab/Fenster geöffnet based on a simple verbal description. We have barely begun to appreciate the magnitude of impact of that capability. Importantly, while early generative AI models are largely proprietary, there are recent efforts Wird in neuem Tab/Fenster geöffnet to create open-access, open-source, multi-language models with liberal intellectual property (IP) rights on both the model and its derivatives. Such open tools will help ensure that the benefits of AI are broadly accessible.
Equally critical for democratization of science will be generating and making available data resources that empower diverse research communities. It has become common to view data as “the new oil Wird in neuem Tab/Fenster geöffnet.” But how do we move beyond a limited set of proprietary “oil wells” to “alternative energy” resources that are more broadly available? I believe that areas of key societal challenges — climate change, renewable energy, sustainable agriculture, poverty, health equity or new therapeutics — could be hugely accelerated by the creation of large, high-quality accessible data resources that can enable broad scientific discovery. A thoughtful and rigorous creation of high-quality data resources is critical here, because data that are biased, faulty or not representative of the complexity of the problem (e.g., the diversity of the human population) could lead us astray Wird in neuem Tab/Fenster geöffnet.
A 25th-anniversary reflection on the Human Genome Project Wird in neuem Tab/Fenster geöffnet provides a valuable perspective on the ingredients necessary to enable projects of this level of impact:
Creating a collaborative, shared resource —moving away from “individual researchers toiling away in isolation to answer a small set of scientific questions” and “focusing instead on the discovery of fundamental information that would inform many follow-on investigations.”
Sharing highly open data, including “policies that shortened the time between the generation and release of data.”
Planning for data analysis from the start.
Fostering the creation and scaling of enabling technical innovations.
Proactively addressing broader societal and ethical implications.
Being audacious, quantitative and flexible in setting project goals, with a strong grounding in “explicit milestones, quality metrics and assessments” and a “willingness to iterate plans as needed.”
These guidelines could and should serve as a North Star in developing a new constellation of open-access, community-led, “big science” efforts to create technologies and data sets in key challenge areas. As discussed above, these initiatives can be implemented effectively via collaborative, community-driven efforts: a miracle of the commons Wird in neuem Tab/Fenster geöffnet. While not inexpensive, we have seen that these efforts can easily pay for themselves many times over in terms of economic, scientific and societal benefits. Done right, these projects will enable a dramatically larger set of researchers to contribute their energy, enthusiasm and intellect toward these areas, and will help generate new and impactful solutions towards some of the most pressing societal problems.
Daphne Koller, PhD
Dr Daphne Koller is CEO and Founder of insitro, a machine learning-driven drug discovery and development company transforming the way drugs are discovered and delivered to patients. She was the co-founder, co-CEO and President of online education platform Coursera, the largest platform for massive open online courses (MOOCs), which has reached over 130 million learners worldwide. She is also the co-founder of Engageli, an interactive digital learning platform aimed at improving learning outcomes.
Daphne was the Rajeev Motwani Professor of Computer Science at Stanford University, where she served on the faculty for 18 years, and where she remains an Adjunct Faculty member. She was formerly Chief Computing Officer of Calico, an Alphabet company in the healthcare space. Daphne received her BSc and MSc from the Hebrew University of Jerusalem and her Phd from Stanford University. She is the author of over 300 refereed publications appearing in venues such as Science, Cell, Nature Genetics, NeurIPS, and ICML, with an h-index of over 145. She is also the co-author of “Probabilistic Graphical Models: Principles and Techniques”, a leading Machine Learning textbook.
Daphne was recognized as one of Time Magazine’s 100 most influential people in 2012 and Newsweek’s 10 most important people in 2010. She has been honored with multiple awards and fellowships during her career including the Sloan Foundation Faculty Fellowship in 1996, the ONR Young Investigator Award in 1998, the Presidential Early Career Award for Scientists and Engineers (PECASE) in 1999, the IJCAI Computers and Thought Award in 2001, the MacArthur Foundation Fellowship in 2004, the ACM Prize in Computing in 2008, the ACM AAAI Allen Newell Award in 2019, the IEEE CS Women of ENIAC Computer Pioneer award and the AnitaB.org Technical Leadership Abie Award Winner in 2022.
Daphne was inducted into the National Academy of Engineering in 2011 and elected to the National Academy of Sciences in 2023; she was elected a fellow of the American Association for Artificial Intelligence in 2004, the American Academy of Arts and Sciences in 2014 and of the International Society of Computational Biology in 2017. Her teaching was recognized via the Stanford Medal for Excellence in Fostering Undergraduate Research, and as a Bass University Fellow in Undergraduate Education.
Mitwirkende*r

DKP