Drug repurposing: Approaches and methods
Drug repurposing can significantly reduce timelines and cost compared with de novo drug development. Explore key considerations below.
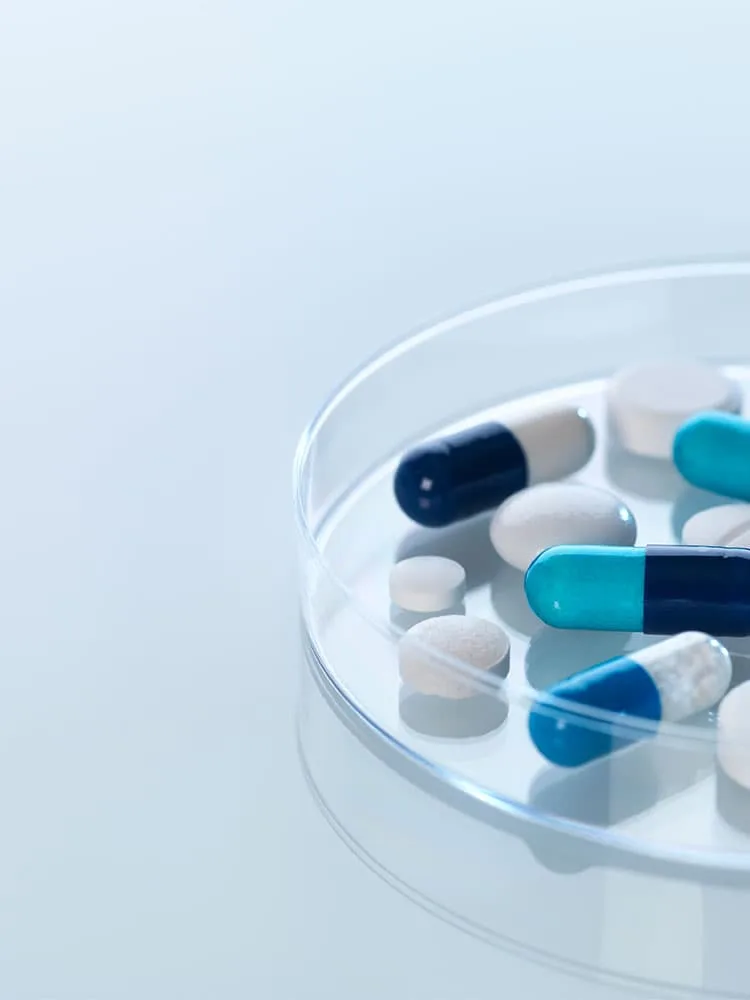
What is drug repurposing?
Drug repurposing, also called drug repositioning or drug reprofiling, is the identification of new therapeutic uses for existing or investigational drugs. It is particularly useful where traditional de novo drug development is not cost-effective, or a cure is urgently needed, such as in the search for a COVID-19 treatments.
The goal of drug repurposing is to quickly identify compounds with an established safety profile and known therapeutic advantages that may prove efficacious for other indications. Pharmaceutical companies are undertaking drug repurposing projects for rare diseases, oncology, infectious and autoimmune diseases and more.
The content on this page outlines key considerations in drug repurposing.
Three main approaches to drug repurposing
Pharmaceutical organizations typically take one of three approaches to drug repurposing as part of a systematic strategy: drug, disease and target centric. They each explore the relationships between drugs, diseases and targets in different ways based on the therapeutic action of a drug. Disease- and target-centric approaches are the most commonly used approaches Wird in neuem Tab/Fenster geöffnet.1
Drug centric
A drug-centric approach expands the application of an existing drug to a new indication. Drug-centric repurposing may begin by:
Discovering off-label use of an approved drug for a new patient population or medical condition outside the scope of a medicine’s existing license or patent
Reviewing investigational or abandoned drugs that initially showed poor efficacy for another indication or did not secure regulatory approval
Identifying new uses for drugs pulled from circulation due to safety or post-market issues that are still efficacious for other medicinal uses
Repositioning drugs that have reached the end of the patent exclusivity period and have generic competitors for new conditions
Disease centric
A disease-centric approach matches diseases with no treatments or with partially effective treatments with approved or failed compounds with therapeutic impact. It is particularly valuable in drug repurposing efforts for rare diseases. It involves identifying diseases with homologous underlying biological mechanisms to the indication the original drug treats. For example, a drug developed to treat cancer could also treat other diseases with uncontrolled cell growth, such as psoriasis.
Target centric
A target-centric approach matches a new indication without a treatment, with an established drug and its known target; the old and new indications typically differ quite significantly. It involves investigating the specific molecular targets that are implicated in the pathology of a disease and using the existing drug proven to modulate those targets. This approach is also particularly useful when seeking to repurpose drugs to treat rare diseases.
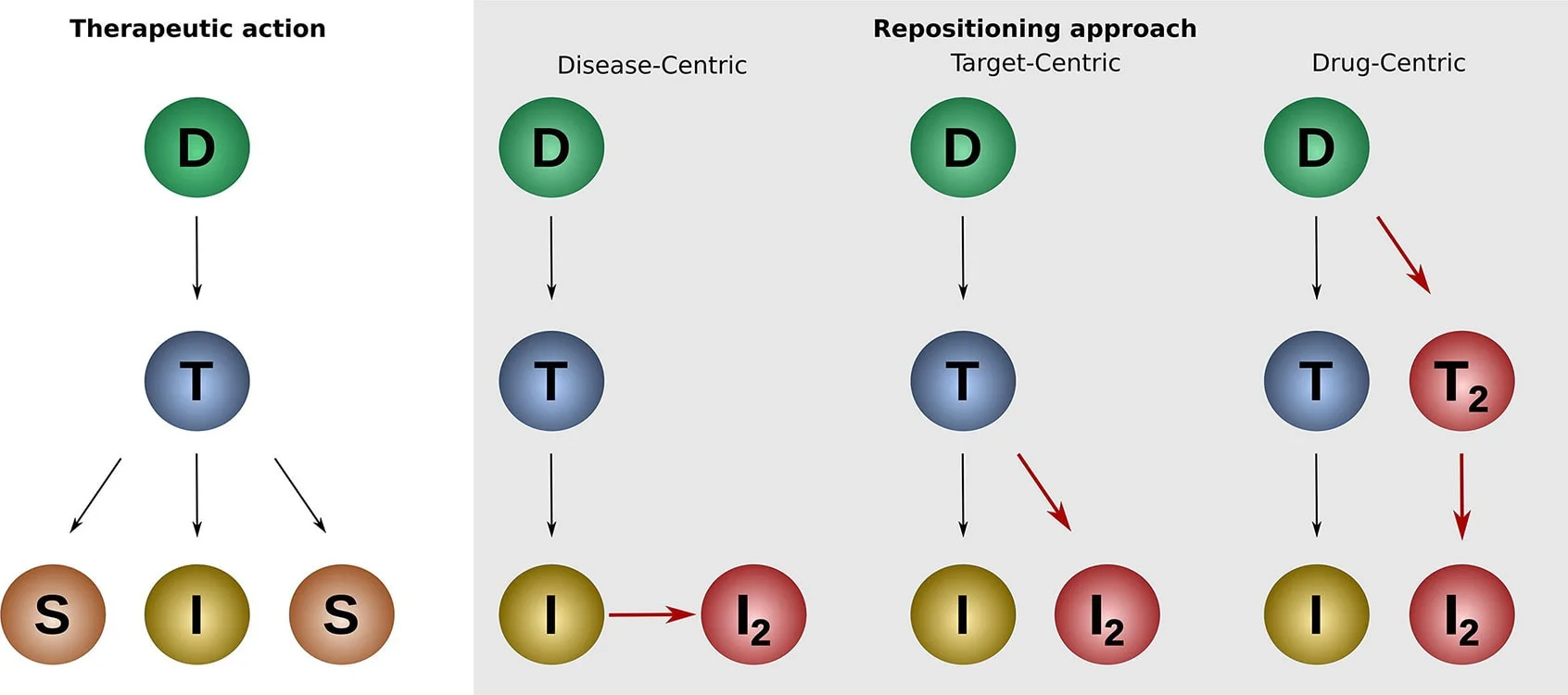
Source: https://www.sciencedirect.com/science/article/pii/S2001037019305021
Key
D – Drug, T – Target, I – Indication, S – Side effect, I2 – New indication, T2 – New Target
Key questions in a drug repurposing project
Whichever repurposing approach a pharmaceutical organization is following, researchers must answer key questions to inform their projects. Accurately answering these questions enables researchers to prioritize candidates with the highest likelihood of a positive outcome and improves the chances of securing market authorization for a drug repurposing candidate.
Important drug repurposing questions include:
What is the molecular target or pathway implicated in the disease of interest?
What existing drugs modulate that target or pathway in the disease of interest?
Does the drug repurposing candidate show significant or improved efficacy over existing therapies?
Is there enough clinical evidence to support the use of the drug in a new context?What potential safety and toxicity risks, and side effects and adverse events can be predicted?
Will the dosage, preparation and duration of treatment need to be adjusted for a new indication?
Is there a sufficient patient population and market size to ensure repurposing is commercially viable?
Does the repurposing candidate have sufficient IP protection and what patents of interest exist?
What evidence is required to support the specific regulatory pathway of a repurposing candidate?
Can the repurposed drug be manufactured and distributed in a cost-effective manner?
To answer these questions accurately, pharmaceutical organizations must consult and analyze a wide range of data sources. These sources and data types can include:
Disease pathology, omics data, scientific literature
Clinical trial data, electronic health records
Drug compound databases
Drug safety data, FAERS data
Pharmacology data, Pk data, MET data, drug interactions
Patents
Regulatory documents
Drug repurposing methods
Advances in data science and computational research enable a range of methodologies that pharmaceutical companies can employ in drug repurposing.
Build a disease model for pathway analysis
Disease pathway analysis gives researchers a deep understanding of underlying disease mechanisms to match repurposing candidates that address those mechanisms. It involves building a disease model – an investigational framework of key biological pathways and networks implicated in a disease, such as molecular and cellular interactions associated with disease biology. Types of data used in creating a model may include gene expression data, protein-protein interaction data, and insights from scientific literature.
The disease model enables researchers to summarize and analyze the underlying biology of disease progression and drug responsiveness. With these insights, researchers can develop and confirm experimental hypotheses for drug repurposing projects.
Create a knowledge graph
Knowledge graphs organize complex biological data into a network. They allow researchers to quickly identify, visualize, and analyze direct and indirect relationships between biological concepts and entities (e.g., disease, cell process) and molecular classes (e.g., protein, small molecules). Armed with these relationship insights, a user can understand disease biology faster and decide what repurposing targets to pursue.
Sophisticated knowledge graphs also capture polarity and effect of the relation (e.g., Protein A negatively regulates Disease X), and may group relationships into specific categories. Different knowledge graphs can represent different areas of study and will therefore include varying data types. For example, a biology knowledge graph could include:
Gene expression — expression, promotor binding, miRNA effect
Proteomics/physical interaction — direct regulation, protein modification, binding
Biomarkers — biomarker, genetic change, quantitative change, state change
Metabolomic/molecular transport + modification — molecular synthesis, molecular transport, chemical reaction
Functional association – between disease and a cellular process or another disease
Regulation – this least specific type of relationship is used if no more specific information is available
A knowledge graph is then overlayed with a user interface that allows researchers to visualize the comprehensive landscape of biological relationships. This allows researchers to gain a rapid understanding of disease biology and focus on critical evidence to power repurposing projects.

Initial view in EmBiology showing upstream and downstream relationship, including effect, on the drug target IL4R. EmBiology is powered by Elsevier’s Biology Knowledge Graph.
Build a graph neural network
Graph neural networks Wird in neuem Tab/Fenster geöffnet (GNN) are an emerging research approach that are growing in popularity.2 A GNN is a form of deep learning; an artificial neural network is created to process rich data structures and connect graph data sources. Graph sources are data structures that represent relationships between objects, such as a knowledge graph or disease model. In drug repurposing, GNNs enable researchers to screen large databases Wird in neuem Tab/Fenster geöffnet of approved drugs and predict drug-target interactions to identify possible therapeutics for new indications.3
Screen compound libraries
A compound library(also known as a chemical library) is composed of real and virtual chemical compounds that have drug-like properties. Such libraries can contain millions of compounds and store data on chemical properties of compounds, such as structure, purity and quantity. Libraries can be broad and diverse, or curated around a specific target or disease. Pharmaceutical companies typically can access proprietary, commercial compound libraries; external libraries via third-party sources, e.g., Reaxys and publicly available libraries such as PubChem.
Using high-throughput screening, researchers can power systematic and large-scale drug repurposing research. This involves screening chemical compounds in a library against multiple biological assays to match compounds with the required biological activity. Such compound focused repurposing seeks to match approved compounds or those that have made it to clinical trial, but no further, with a novel indication.
Automated literature and text mining
Automated literature and text mining is a computational method powered by natural language processing (NLP) and machine learning techniques and is a highly efficient method of searching through a large body of knowledge. Automated literature and text mining enables researchers to rapidly search, filter and understand millions of articles; finding patterns and connections at a volume and speed that would be impossible manually.
It works by extracting data from scientific texts (including published research, patent data and clinical trials data) that pertains to research questions. Automated literature and text mining is beneficial in drug repurposing because scientific literature contains huge volumes of data on research and clinical studies that can help shed light on alternative applications for approved or failed drugs. Researchers can “mine” for specific statements, such as what entity causes or worsens a disease or symptoms associated with an indication. Results can then be combined with a scoring mechanism to identify the most promising drug repurposing candidates.
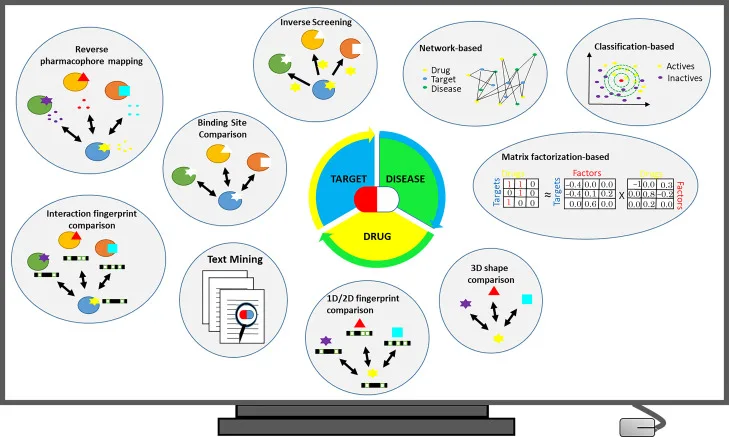
Overview of commonly used in silico drug repurposing strategies. Source: “Current In Silico Drug Repurposing Strategies Wird in neuem Tab/Fenster geöffnet," Systems Medicine, Volume 2, 2021.
In silico modeling and digital twins are computational approaches that enable researchers to create virtual representations of biological systems, indications and patients to simulate experiments and predict responses under different conditions. Models are created using numerous high-quality sources including scientific literature, clinical data and electronic health records (EHRs), and regulatory and approval datasets. They are built on a range of parameters specific to the indication of interest or patient population, including certain physical, genetic and medical characteristics.
Through these methods, researchers can simulate the impact a repurposed drug would have on the body of a patient living with a particular condition and predict the effectiveness of an existing drug against a new indication. Using a “digital patient” is a quick and safe way to predict repurposing options and build drug-specific exposure models that allow the accurate forecasting of pharmacokinetic properties with no risk to real patients.
What are the benefits of drug repurposing?
Developing new therapeutics for unmet medical needs is a critical global challenge. Drug discovery and design can take 10 to 15 years and cost up to $2.6 billion Wird in neuem Tab/Fenster geöffnet for a drug to reach the market. Drug repurposing can significantly alter that timeline and cost, while uncovering treatments for many diseases that lack effective therapeutics today.
Drug repurposing can increase ROI on existing assets, extending the revenue potential of portfolio drugs and mitigating patent expiry. It also offers new avenues of hope for patients living with untreated or difficult-to-treat conditions, especially rare and neglected diseases.
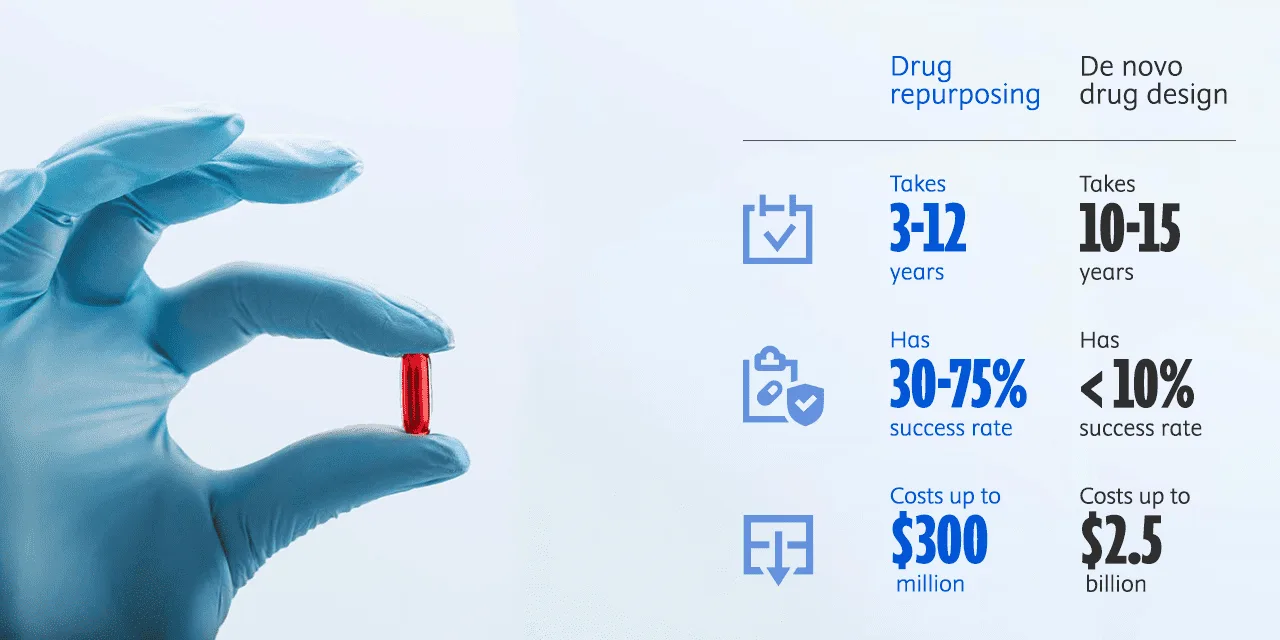
Data source: https://doi.org/10.1016/j.csbj.2020.04.004
Notable cost and time savings
Compared to traditional development, drug repurposing offers considerable time and cost savings Wird in neuem Tab/Fenster geöffnet:
… repurposed drugs are generally approved sooner (3–12 years) and at reduced (50–60%) cost. In addition, while ~10% of new drug applications gain market approval, approximately 30% of repurposed drugs are approved, giving companies a market-driven incentive to repurpose existing assets.
New treatments for rare diseases
There is no licensed treatment or cure available for 95% of rare diseases Wird in neuem Tab/Fenster geöffnet.4 Therefore, repurposing offers a viable alternative to new drug discovery when candidates that have proven safe in either preclinical models or clinical human usage also show efficacy for a rare disease and can be repositioned for the new indication.
Rapid response to emerging needs
Drug repurposing is beneficial during health emergencies to quickly identify potential treatments. During the COVID-19 pandemic, several drugs were repurposed under emergency authorization, including Remdesivir Wird in neuem Tab/Fenster geöffnet5, an antiviral developed as a treatment for Ebola to reduce virus replication and Dexamethasone Wird in neuem Tab/Fenster geöffnet6, a widely used steroid that reduces mortality in hospitalized patients requiring ventilation.
Less or no animal testing required
Animal testing provides researchers with insights to understand how a human body processes a drug and how the drug interacts with the body. When repurposing drugs, these pharmacokinetics and pharmacodynamics insights are already known, so animal testing carried out in preclinical phases is eliminated.
Drug repurposing challenges
Technical challenges to drug repurposing
There are several technical challenges that pharmaceutical organizations may face when pursuing computational methods to support drug repurposing projects.
Data volume and hygiene
Drug repurposing requires access to considerable volumes of data, including compound libraries, patent data, pharmacological data, and published scientific literature. Large datasets are more likely to suffer from poor data hygiene, increasing the likelihood of errors, inconsistencies and duplications. Some datasets may be restricted by privacy and security concerns, regulatory rules, or are inaccessible externally. Yet missing data impacts outcomes.
Data heterogeneity
The types of data required to support drug repurposing are varied and originate from multiple sources. Data are often siloed and stored in multiple formats, such as unstructured text files and images, electronic lab notebooks, spreadsheets and databases. Repurposing also uses many data types, such as biology/omics and chemistry data. The heterogeneity of the data poses significant challenges for integration, analysis and management.
Tooling and platform barriers
Significant and highly scalable computing power is needed to collect, store, process, manage and analyze data as volumes expand and the number of sources increase. Platforms that can easily connect data via APIs are essential to “serve” data to internal audiences who are not data science experts. Specialist techniques to organize data are also important, for example, expert ontologies, taxonomies, indexing and metadata tagging.
Lack of technical expertise
Building a knowledge graph or graph neural network, or creating an in silico model or digital twin, requires cross-domain expertise that spans science and technology. Smaller to medium-sized pharmaceutical organizations may not have teams capable of both integrating and harmonizing fragmented and disparate datasets, and who can parse a drug repurposing research question with access to the full context of the question.
Efficacy and safety
A repurposed drug candidate may prove to be less effective for a new indication than it was for the original licensed purpose, or it may not show a marked increase in efficacy over treatments that already exist. In some cases, there may be limited clinical evidence to support the use of the new indication being pursued.
Additionally, drug repurposing candidates may not be as beneficial when newly employed in combination therapies than in their previous usage as a single therapy. Combining drugs also necessitates new clinical trials; expensive and time-consuming clinical studies may then negate the cost savings of a repurposed drug. Where a generic equivalent for the drug exists, the subsequently lower potential profit margin also does not support the business case for clinical trials.
Regulatory challenges to drug repurposing
As in de novo development, securing marketing authorization for repurposed drugs from regulatory agencies like the FDA, EMA and MHRA can be a barrier. While typically, existing drugs do have an easier path to approval for new indications because of their known safety profile and established use in humans, challenges still occur.
While preclinical testing is eliminated in repurposing projects, clinical trials are still required in most scenarios to demonstrate efficacy and safety in the target indication. The extent of the trials required varies depending on what data are available on the drug and the nature of the repurposing project. For instance, there are more stringent rules around drugs for children. Moreover, in rare diseases, difficulties with finding enough patients in the target cohort to conduct a statistically significant trial may halt a project completely.
Human clinical trials are the most expensive research phase, and the cost may outweigh the potential return on investment from a repurposed asset. It can also be harder to obtain reimbursement from payers because reimbursement codes (used by insurance companies in healthcare billing and claims) may not apply for a new use, which will also limit adoption in practice.
Drug repurposing and intellectual property rights
Securing new patent applications or licensing agreements for a repurposed drug can be complex and time-consuming. Pharmaceutical organizations need to be sure of the commercial viability of a project; they also need access to the latest information on existing IP and patent protections before applying for a new patent or a patent expansion. If IP protection is limited, they may not be able to patent the drug for a new use and recoup any further investment.
Timing can also be a challenge. If a pharmaceutical organization has a drug whose patent is expiring, they can extend the patent protection if they discover a new or extended indication. But the repurposing project and the patent application must begin early enough so that the current patent does not end, and exclusivity is not lost. One study Wird in neuem Tab/Fenster geöffnet7 found that the nature of expiring exclusivity negatively impacts the discovery of secondary indications. Of 197 new drugs approved by the FDA between July 1997 and May 2020, there was found to be a near-zero probability of a new indication being added 15 years after FDA approval.
Examples of drug repurposing
Non-cancer drugs that target a homologous underlying biological mechanism or mutation can be repurposed to treat cancer. For example, Raloxifene was originally developed as a treatment for osteoporosis but has been used to reduce the risk of breast cancer in high-risk women. Alternatively, an existing cancer drug can be used to treat cancer in a different organ if it is caused by the same mutation or disruption of the same mechanism. For example, Tamoxifen was originally developed as a treatment for breast cancer but has been used to treat other types of cancer, such as ovarian and endometrial.
Learn more about drug repurposing in oncology through this collaborative project Wird in neuem Tab/Fenster geöffnet between Elsevier and the Sinergia Consortium, focusing on diffuse intrinsic pontine glioma (DIPG), a rare form of childhood brain cancer.
During the COVID-19 pandemic, several drugs were repurposed under emergency authorization, including Remdesivir, an antiviral developed as a treatment for Ebola to reduce virus replication, and Dexamethasone, a widely used steroid that reduces mortality in hospitalized patients requiring ventilation. In silico modelling can be used to accelerate drug repurposing and rapidly simulate the efficacy and interactions of drugs. An Elsevier and ExactCure study identified 20 candidates for repurposing by leveraging compounds known to disrupt coronavirus-induced cytokine storm using this approach.
Successful examples of repurposing for rare diseases often stem from collaborative approaches that leverage both scientific, data science, computational and technical expertise. For example, a datathon run by Elsevier and The Pistoia Alliance identified four new candidates for drug repurposing to treat chronic pancreatitis. And a demonstration by SciBite and Stardog that took just 40 minutes showed how to build a knowledge graph to identify repurposing candidates for Friedrich’s Ataxia.
Learn more about repurposing for rare diseases through the Elsevier Year of the Zebra Wird in neuem Tab/Fenster geöffnet initiative.
Studying a drug's mechanism of action and evaluating its safety and efficacy from preclinical animal studies, and from veterinary medicine, can indicate potential for use in humans. Through this approach, drugs used in animal care may be repurposed in humans following further testing. For example, miltefosine, a canine antiparasitic agent, was found to be an effective treatment for leishmaniasis, a parasitic infection in humans. The reverse approach is also applicable; gabapentin, developed as an anticonvulsant for humans, has been used to treat chronic pain in animals, and trazodone, used for depression and anxiety in humans, is also prescribed for canine anxiety.
A less known but growing area of research is the repurposing of human drugs in agriculture, specifically in the protection of crops. For example, exploring insights from anti-parasitic drug discovery may offer avenues for researchers engaged in the development of fungicides to protect crops. A team of Elsevier researchers produced a proof-of-concept supporting this approach for the malaria-causing parasite Plasmodium falciparum.
Bioactivity data and literature were mined and extracted from literature and patents to find compounds acting against Plasmodium falciparum targets to then look at similar fungal targets and suggest that these compounds may be active against those.
Read more about drug repurposing
References
Daniele Parisi, Melissa F. Adasme, Anastasia Sveshnikova, Sarah Naomi Bolz, Yves Moreau, Michael Schroeder, Drug repositioning or target repositioning: A structural perspective of drug-target-indication relationship for available repurposed drugs, Computational and Structural Biotechnology Journal, Volume 18, 2020, Pages 1043-1055. https://www.sciencedirect.com/science/article/pii/S2001037019305021
Abdalsamad Keramatfar, Mohadeseh Rafiee, Hossein Amirkhani, Graph Neural Networks: A bibliometrics overview, Machine Learning with Applications, Volume 10, 2022. https://www.sciencedirect.com/science/article/pii/S2666827022000780
Siddhant Doshi, Sundeep Prabhakar Chepuri, A computational approach to drug repurposing using graph neural networks, Computers in Biology and Medicine, Volume 150, 2022. https://www.sciencedirect.com/science/article/pii/S001048252200717X
Helen I. Roessler, Nine V.A.M. Knoers, Mieke M. van Haelst, Gijs van Haaften, Drug Repurposing for Rare Diseases, Trends in Pharmacological Sciences, Volume 42, Issue 4, 2021. https://www.sciencedirect.com/science/article/pii/S0165614721000067
Revaz Metchurtchlishvili, Nikoloz Chkhartishvili, Akaki Abutidze, Marina Endeladze, Marine Ezugbaia, Ana Bakradze, Tengiz Tsertsvadze, Effect of remdesivir on mortality and the need for mechanical ventilation among hospitalized patients with COVID-19: real-world data from a resource-limited country, International Journal of Infectious Diseases, Volume 129, 2023, Pages 63-69. https://www.sciencedirect.com/science/article/pii/S120197122300022X
Shu-Min Lin, Chung-Shu Lee, Allen Chung-Cheng Huang, Tzu-Hsuan Chiu, Ko-Wei Chang, Tse-Hung Huang, Tsung-Hsien Yang, Yi-Hsien Shiao, Fu-Tsai Chung, Chyi-Liang Chen, Cheng-Hsun Chiu, Effects of dexamethasone use on viral clearance among patients with COVID-19: a multicenter cohort study, International Journal of Infectious Diseases, Volume 128, 2023, Pages 257-264. https://www.sciencedirect.com/science/article/pii/S1201971223000103
Sahragardjoonegani B, Beall RF, Kesselheim AS, Hollis A. Repurposing existing drugs for new uses: a cohort study of the frequency of FDA-granted new indication exclusivities since 1997. J Pharm Policy Pract. Jan 4, 2021. https://pubmed.ncbi.nlm.nih.gov/33397471/